How has big data changed the world of business? The answer is profound and multifaceted. From revolutionizing customer understanding to optimizing supply chains and automating operations, the impact of big data is undeniable. This exploration delves into the transformative effects of leveraging massive datasets, revealing how businesses are using data-driven insights to make smarter decisions, enhance efficiency, and gain a competitive edge in today’s dynamic marketplace. We’ll examine real-world examples, explore ethical considerations, and uncover the potential for future innovations driven by this powerful resource.
Enhanced Decision-Making
Big data analytics has fundamentally reshaped business decision-making, shifting from gut feelings and limited data to data-driven strategies informed by vast datasets and sophisticated analytical techniques. This transformation allows businesses to understand customer behavior, market trends, and operational inefficiencies with unprecedented clarity, leading to more informed and effective decisions.
Big data analytics empowers businesses to move beyond reactive measures to proactive strategies. Instead of responding to problems as they arise, companies can anticipate potential challenges and opportunities, enabling them to optimize resource allocation, mitigate risks, and capitalize on emerging market trends. This proactive approach significantly improves profitability and competitive advantage.
Examples of Big Data-Driven Decision-Making
Several companies have successfully harnessed the power of big data for strategic decision-making. These examples highlight the diverse applications of big data analytics across various industries and the significant impact it can have on business outcomes. The effectiveness stems from the combination of appropriate data sources and the analytical methods used to extract actionable insights.
Netflix’s Content Recommendation System
Netflix utilizes vast amounts of user data, including viewing history, ratings, search queries, and even the time spent watching particular scenes, to power its recommendation engine. This system employs collaborative filtering and machine learning algorithms to predict which shows and movies users are most likely to enjoy. This data-driven approach has significantly increased user engagement and retention, directly impacting subscriber growth and revenue. The data used includes user viewing habits, ratings, and metadata about the content itself. The analytical methods employed include collaborative filtering, content-based filtering, and machine learning algorithms.
Walmart’s Supply Chain Optimization
Walmart leverages big data to optimize its massive supply chain. By analyzing sales data, weather patterns, and local economic indicators, Walmart can predict demand fluctuations and adjust inventory levels accordingly. This precise inventory management minimizes waste, reduces storage costs, and ensures products are available when and where they’re needed. The data used includes sales data, weather data, economic indicators, and logistical data. The analytical methods include predictive modeling, time series analysis, and optimization algorithms.
American Airlines’ Revenue Management
American Airlines uses big data analytics to optimize its pricing and revenue management strategies. By analyzing historical flight data, passenger demographics, and competitor pricing, the airline can dynamically adjust ticket prices to maximize revenue. This data-driven approach ensures optimal pricing across different routes and passenger segments, leading to improved profitability. The data used includes historical flight data, passenger demographics, competitor pricing, and market demand data. The analytical methods include predictive modeling, regression analysis, and optimization algorithms.
Comparison of Traditional and Big Data-Driven Decision-Making
Company Name | Decision Type | Data Used | Outcome |
---|---|---|---|
Netflix | Content Recommendation | User viewing history, ratings, search queries | Increased user engagement and retention |
Walmart | Supply Chain Optimization | Sales data, weather patterns, economic indicators | Minimized waste, reduced costs, improved availability |
American Airlines | Revenue Management | Flight data, passenger demographics, competitor pricing | Improved profitability, optimized pricing |
Traditional Approach (Example: Retail Store) | Inventory Management | Sales figures (limited data), gut feeling | Potential overstocking or stockouts, inefficient resource allocation |
Improved Customer Understanding
Big data has fundamentally reshaped how businesses understand and interact with their customers. The sheer volume, velocity, and variety of data now available provide unprecedented insights into customer behavior, preferences, and needs, leading to more effective marketing, improved customer service, and ultimately, increased profitability. This shift is largely driven by advancements in data collection, storage, and analytical capabilities, allowing companies to move beyond traditional, often limited, CRM approaches.
The revolution in customer relationship management (CRM) facilitated by big data is characterized by a move from reactive to proactive strategies. Instead of simply responding to customer inquiries, businesses can now anticipate needs and tailor their offerings accordingly. This predictive capability allows for personalized experiences that foster loyalty and drive sales. The availability of real-time data also enables immediate responses to customer feedback, enhancing the overall customer journey and building stronger relationships.
Big Data’s Role in Personalizing Customer Experiences
Companies leverage big data in numerous ways to personalize customer experiences. For example, e-commerce giants like Amazon utilize purchase history, browsing behavior, and product ratings to recommend relevant items, significantly increasing sales conversion rates. Netflix similarly uses viewing history and user ratings to suggest movies and TV shows, optimizing user engagement and satisfaction. Furthermore, many companies use big data to personalize email marketing campaigns, tailoring content and offers to specific customer segments based on their demographics, purchase patterns, and online activity. This level of personalization creates a more engaging and relevant customer experience, improving customer satisfaction and driving brand loyalty. For instance, a clothing retailer might send personalized email recommendations based on past purchases and browsing history, showcasing items likely to appeal to individual customer preferences.
Ethical Considerations in Customer Profiling and Targeting
The use of big data for customer profiling and targeting raises significant ethical concerns. The collection and analysis of vast amounts of personal data can lead to privacy violations if not handled responsibly. Issues of data security, transparency, and consent are paramount. For example, the use of behavioral tracking and targeted advertising can feel intrusive to customers if they are unaware of the extent of data collection. Furthermore, biases in algorithms used for customer profiling can lead to discriminatory outcomes, reinforcing existing inequalities. Striking a balance between leveraging the benefits of big data for personalized marketing and protecting customer privacy is a crucial challenge that companies must address. Robust data governance policies, transparent data collection practices, and mechanisms for customer control over their data are essential to mitigate these risks.
Hypothetical Customer Segmentation Strategy
A hypothetical customer segmentation strategy using big data could divide customers into four segments: High-Value Loyalists (high purchase frequency, high average order value, high engagement), At-Risk Customers (decreasing purchase frequency, declining average order value, low engagement), Potential High-Value Customers (high browsing activity, high average order value of initial purchases, but low purchase frequency), and Price-Sensitive Customers (low average order value, high purchase frequency, sensitive to discounts and promotions). These segments would be identified through analysis of transactional data, website activity, social media interactions, and other relevant data sources. Marketing strategies would then be tailored to each segment. For example, High-Value Loyalists might receive exclusive offers and personalized recommendations, while At-Risk Customers might receive targeted retention campaigns. Potential High-Value Customers could be encouraged to make repeat purchases through targeted promotions, and Price-Sensitive Customers could be targeted with discounts and value-oriented promotions. This targeted approach ensures that marketing resources are allocated efficiently and effectively, maximizing ROI.
Operational Efficiency and Automation: How Has Big Data Changed The World Of Business
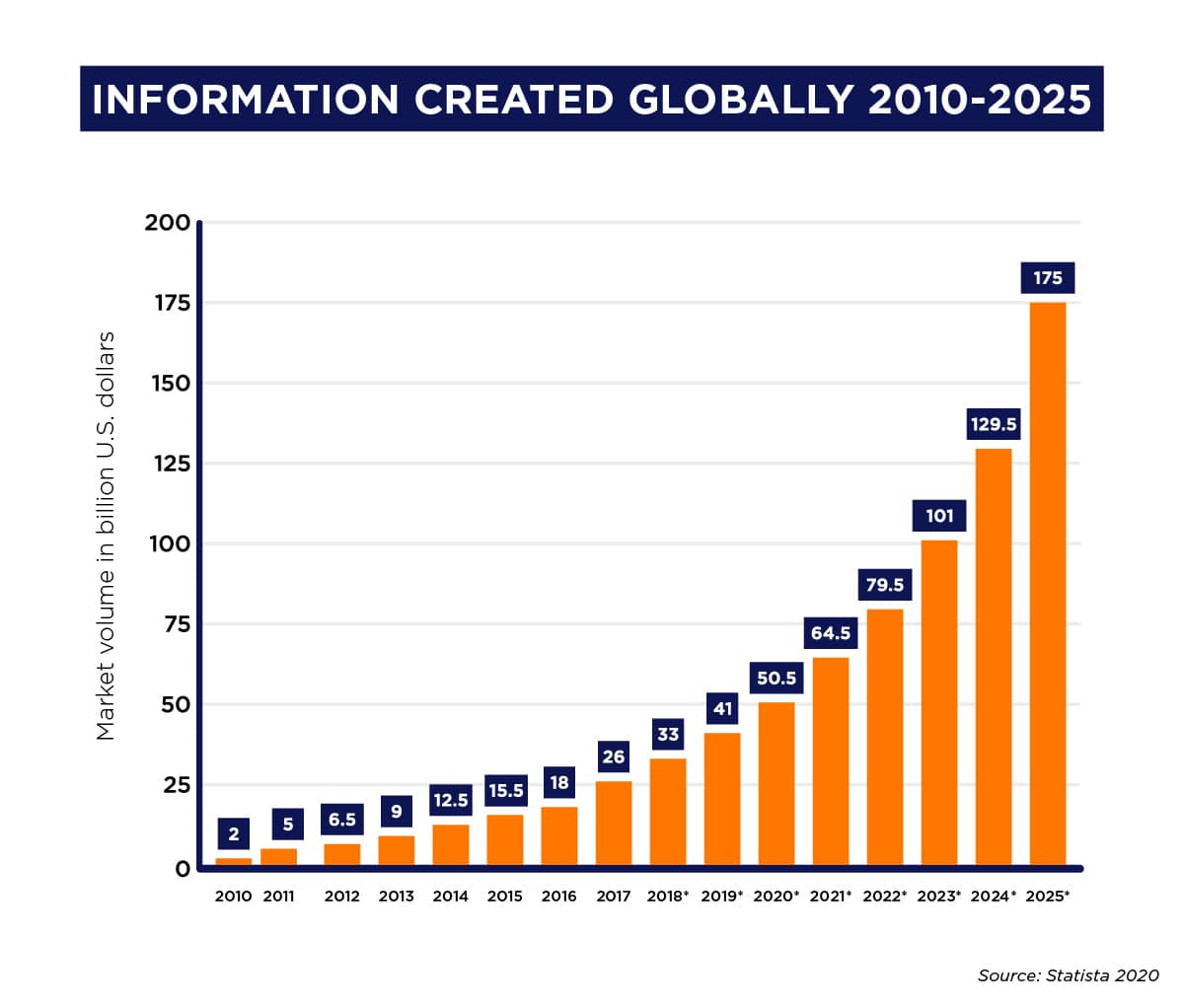
Big data’s impact extends far beyond improved decision-making and customer understanding; it’s fundamentally reshaping business operations, driving unprecedented levels of efficiency and automation. The ability to collect, analyze, and interpret massive datasets allows companies to streamline processes, predict potential issues, and automate tasks previously handled manually, leading to significant cost savings and increased productivity.
Big data analytics fuels automation by identifying patterns and trends that inform the development of intelligent systems. These systems can then autonomously execute tasks, optimize resource allocation, and proactively address operational challenges, resulting in a leaner, more responsive, and profitable organization. This shift towards automation is not merely about replacing human workers; it’s about empowering employees to focus on higher-value activities, fostering innovation and strategic growth.
Automation Examples Across Industries
Big data-driven automation is transforming diverse sectors. In manufacturing, predictive maintenance uses sensor data to anticipate equipment failures, minimizing downtime and reducing repair costs. For example, a General Electric wind turbine uses sensors to monitor its components and predict potential failures. This allows for proactive maintenance, preventing costly repairs and maximizing operational uptime. In the financial services industry, algorithmic trading leverages real-time market data to execute trades at optimal prices, increasing profitability and reducing risk. Fraud detection systems analyze transaction patterns to identify suspicious activities, protecting both businesses and customers from financial losses. Supply chain management benefits from big data analytics by optimizing logistics, predicting demand fluctuations, and streamlining inventory management. For instance, Amazon uses big data to forecast demand for products, optimizing its warehouse operations and ensuring timely delivery to customers.
Cost-Effectiveness of Big Data-Driven Automation
While the initial investment in big data infrastructure and analytics capabilities can be substantial, the long-term cost savings often outweigh the upfront expenses. Traditional methods often rely on manual processes, which are prone to errors, inefficient, and costly in terms of labor and time. Big data-driven automation, in contrast, reduces operational costs by automating repetitive tasks, minimizing errors, and optimizing resource allocation. Consider the example of a call center: using big data analytics to predict customer service needs and automate responses can significantly reduce the number of agents required, leading to substantial cost savings. The return on investment (ROI) for big data-driven automation varies depending on the industry and specific application, but numerous case studies demonstrate its significant cost-effectiveness.
Operational Areas Significantly Impacted by Big Data
Big data has revolutionized numerous operational areas. The impact is most profound in:
- Supply Chain Management: Optimizing logistics, predicting demand, improving inventory management.
- Customer Service: Automating responses, personalizing interactions, improving response times.
- Manufacturing: Predictive maintenance, optimizing production processes, reducing waste.
- Risk Management: Fraud detection, credit scoring, predicting financial risks.
- Human Resources: Talent acquisition, employee engagement, performance management.
New Product and Service Development
Big data has revolutionized the process of new product and service development, empowering businesses to move beyond intuition and guesswork towards data-driven innovation. By analyzing vast quantities of structured and unstructured data, companies can identify previously unseen market trends, understand customer needs with unprecedented accuracy, and ultimately develop products and services that resonate strongly with their target audiences. This data-driven approach minimizes the risk of costly failures and accelerates the time-to-market for successful innovations.
The ability to identify new market opportunities and develop innovative products is significantly enhanced through the use of big data analytics. Businesses can leverage various techniques to gain actionable insights. For example, analyzing demographic data, purchase history, and web browsing behavior can reveal untapped market segments and unmet customer needs. Furthermore, analyzing social media trends and online reviews can provide valuable feedback on existing products and services, highlighting areas for improvement and suggesting new product features.
Identifying Untapped Market Opportunities
Big data analysis allows businesses to identify previously unknown market segments and opportunities. By correlating various data points, companies can discover hidden relationships and patterns that indicate potential demand for new products or services. For example, a retailer might analyze purchase data to identify a previously unrecognized customer segment with specific preferences, leading to the development of a new product line tailored to their needs. Similarly, a streaming service might use viewing habits and demographic data to identify a niche audience interested in a particular type of content, prompting the creation of original programming targeted at that segment. This proactive approach to market research reduces the risk associated with launching new products into an uncertain market.
Predictive Analytics in New Product Development
Predictive analytics plays a crucial role in mitigating the risks associated with new product development. By analyzing historical data and applying statistical models, businesses can forecast the potential success or failure of a new product before it is even launched. This allows for informed decision-making, resource allocation, and adjustments to the product’s design or marketing strategy based on predicted outcomes. For instance, a company launching a new mobile app might use predictive analytics to forecast its download rate and user engagement based on similar apps and market trends, allowing them to allocate marketing resources effectively and adjust features based on anticipated user behavior.
Sentiment Analysis and Product Design
Sentiment analysis of social media data provides invaluable insights into customer opinions and preferences. By analyzing the emotional tone of online conversations, businesses can gauge public perception of their existing products and identify areas for improvement in future designs. For example, a car manufacturer might analyze social media posts about its latest model to identify recurring complaints about specific features, leading to design modifications in subsequent models. Furthermore, sentiment analysis can inform marketing strategies, allowing companies to tailor their messaging to resonate with the emotional landscape of their target audience. Analyzing positive and negative sentiment around competing products can also reveal opportunities to differentiate and improve market positioning.
Risk Management and Fraud Detection
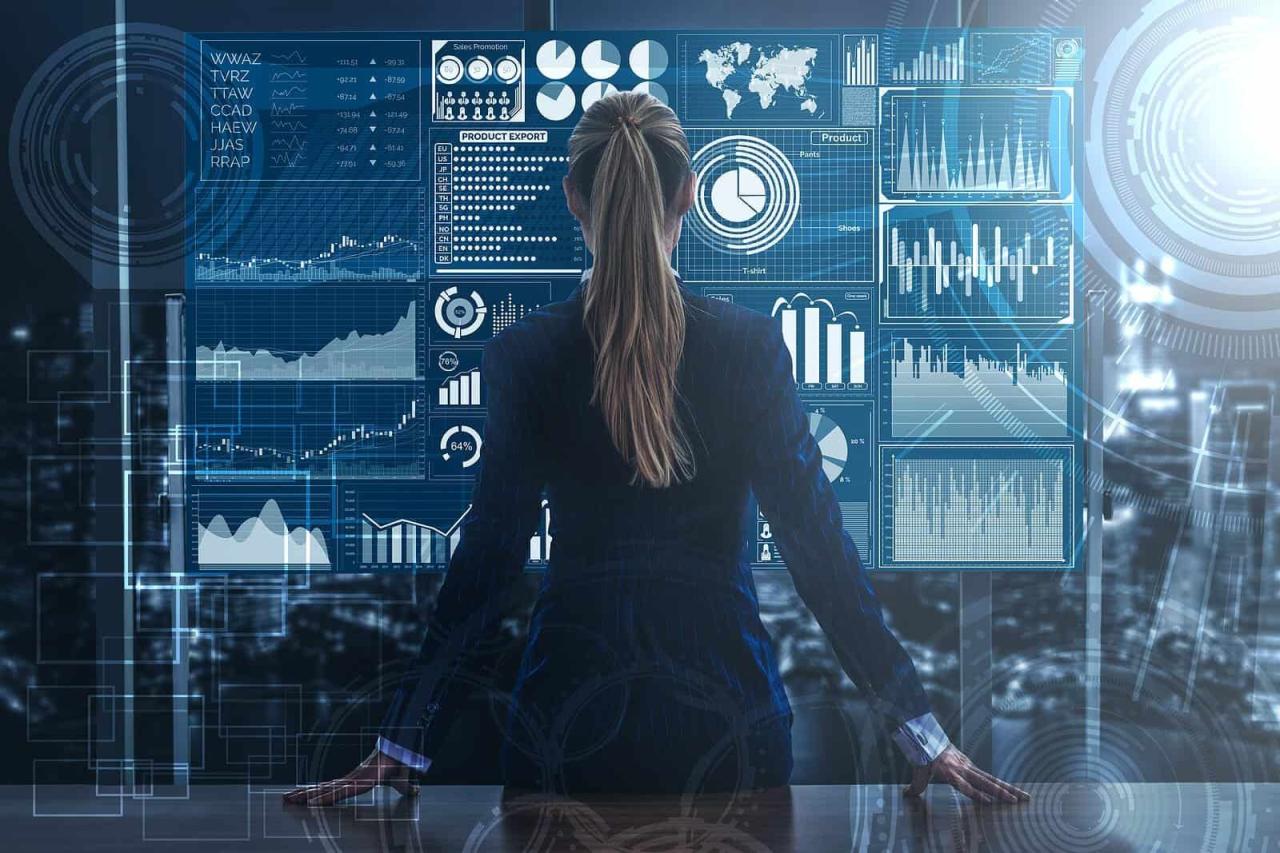
Big data analytics has revolutionized risk management and fraud detection across various industries. The sheer volume, velocity, and variety of data now available allows businesses to identify patterns and anomalies indicative of risk or fraudulent activity far more effectively than traditional methods. This enhanced capability leads to more proactive risk mitigation strategies and more efficient fraud prevention systems.
Big data improves risk assessment and mitigation by providing a more comprehensive view of potential threats. Instead of relying on limited historical data or gut feeling, businesses can leverage massive datasets to identify subtle correlations and predict future risks with greater accuracy. This applies across various contexts, from credit risk assessment in finance to supply chain disruptions in logistics and even predicting equipment failures in manufacturing. By analyzing diverse data sources – customer behavior, market trends, sensor readings, and more – businesses can develop more sophisticated risk models and implement targeted interventions.
Big Data Applications in Fraud Detection
Big data plays a crucial role in detecting and preventing fraud across multiple sectors. For example, in the financial services industry, banks use big data to identify unusual transaction patterns, such as unusually large withdrawals or transfers to unfamiliar accounts, which may signal fraudulent activity. E-commerce companies leverage big data to detect fraudulent transactions by analyzing customer purchase history, IP addresses, and shipping addresses. Insurance companies use big data to identify fraudulent claims by analyzing medical records, claim histories, and social media data. In each case, the scale and complexity of the data involved necessitate advanced analytical techniques.
Algorithms and Techniques in Big Data-Driven Fraud Detection
Several algorithms and techniques are central to big data-driven fraud detection systems. Machine learning, particularly supervised learning techniques like logistic regression and support vector machines, are used to build predictive models that identify fraudulent transactions based on historical data. Unsupervised learning methods, such as clustering and anomaly detection, are employed to identify unusual patterns or outliers that may indicate fraudulent activity. Deep learning models, such as recurrent neural networks (RNNs) and convolutional neural networks (CNNs), can analyze sequential data and complex patterns to detect sophisticated fraud schemes. Furthermore, graph databases and network analysis are used to visualize relationships between entities and identify suspicious connections.
A Typical Big Data Fraud Detection Workflow
Imagine a flowchart. It begins with Data Ingestion, where diverse data sources (transactional data, customer data, sensor data, etc.) are collected and consolidated. This is followed by Data Preprocessing, involving data cleaning, transformation, and feature engineering. Next, Model Training uses historical data to train machine learning models to identify fraudulent patterns. Then, Real-time Scoring applies these trained models to new data to flag potentially fraudulent activities. Finally, Alerting and Investigation triggers alerts for suspicious activities, allowing investigators to review flagged transactions and take appropriate action. This workflow highlights the iterative nature of fraud detection, with continuous model refinement based on feedback and new data.
Supply Chain Optimization
Big data analytics has revolutionized supply chain management, offering unprecedented visibility and control over complex logistical networks. By leveraging vast datasets encompassing everything from raw material sourcing to final product delivery, businesses can identify inefficiencies, predict disruptions, and optimize their operations for greater profitability and resilience. This allows for a more agile and responsive supply chain, better equipped to handle unexpected challenges and capitalize on emerging opportunities.
Big data analytics optimizes supply chain management and logistics through advanced analytical techniques that reveal hidden patterns and insights within operational data. This data-driven approach enables companies to make informed decisions across various stages of the supply chain, from procurement and manufacturing to warehousing and distribution. The ability to analyze real-time data provides a dynamic understanding of inventory levels, transportation routes, and customer demand, allowing for proactive adjustments and improved resource allocation.
Predictive Modeling for Supply Chain Disruptions, How has big data changed the world of business
Predictive modeling, a core component of big data analytics, plays a crucial role in anticipating and mitigating supply chain disruptions. By analyzing historical data, market trends, and external factors like weather patterns or geopolitical events, businesses can build models that predict potential risks with reasonable accuracy. For example, a model might forecast a potential shortage of a specific component based on supplier performance history and anticipated demand. This allows companies to proactively secure alternative suppliers, adjust production schedules, or even preemptively stock up on necessary materials, minimizing the impact of unforeseen circumstances. Companies like Walmart have extensively used predictive modeling to optimize their massive supply chain, predicting demand fluctuations and ensuring timely product availability. Their sophisticated algorithms analyze point-of-sale data, weather forecasts, and social media trends to anticipate consumer behavior and adjust inventory levels accordingly.
Inventory Management and Waste Reduction
A hypothetical scenario illustrates how big data can significantly improve inventory management and reduce waste. Consider a large manufacturing company producing consumer electronics. By analyzing historical sales data, production forecasts, and real-time inventory levels, the company can build a predictive model to optimize its inventory levels. The model can accurately forecast demand for each product based on various factors, including seasonality, marketing campaigns, and economic indicators. This allows the company to reduce excess inventory, minimizing storage costs and the risk of obsolescence. Simultaneously, the model can predict potential stockouts, enabling timely procurement of raw materials and components to prevent production delays. This optimized inventory management strategy not only minimizes waste but also ensures efficient production and timely delivery of products, ultimately improving customer satisfaction and profitability. This approach mirrors the strategies employed by companies like Amazon, who utilize big data to fine-tune their inventory management, ensuring optimal stock levels across their vast network of warehouses.
Examples of Companies Utilizing Big Data for Supply Chain Efficiency
Several companies have demonstrated the transformative power of big data in optimizing their supply chains. Nike, for instance, uses big data to analyze consumer preferences and trends, allowing them to design and manufacture products that precisely meet market demand. This reduces excess inventory and ensures that products are available when and where consumers want them. Similarly, Unilever leverages big data to optimize its logistics network, improving delivery times and reducing transportation costs. Their sophisticated analytics platforms analyze various factors, including weather patterns, traffic conditions, and warehouse capacity, to create efficient delivery routes and minimize delays. These examples highlight the potential for big data to drive significant improvements in supply chain efficiency, profitability, and overall business performance.
Marketing and Sales Enhancements

Big data has fundamentally reshaped marketing and sales strategies, moving the industry from broad, generalized campaigns to highly personalized, data-driven approaches. This shift allows businesses to understand their customers with unprecedented depth, leading to more effective targeting, improved conversion rates, and ultimately, increased revenue. The availability of massive datasets, coupled with advanced analytical tools, has empowered marketers to make informed decisions, optimize campaigns in real-time, and measure the effectiveness of their efforts with greater precision.
Big data’s impact on marketing and sales is multifaceted, influencing everything from customer segmentation and campaign personalization to predictive analytics and fraud detection. This transformation allows businesses to move beyond guesswork and instead rely on concrete data to guide their strategies, resulting in improved ROI and a stronger competitive advantage.
Targeted Advertising Campaigns
Big data fuels the creation of highly targeted advertising campaigns by enabling precise customer segmentation based on a wide array of factors including demographics, online behavior, purchase history, and even social media activity. This granular level of understanding allows marketers to deliver the right message to the right person at the right time, maximizing engagement and minimizing wasted ad spend. For example, a clothing retailer might use big data to identify customers interested in sustainable fashion, then target them with ads featuring eco-friendly products. Similarly, a financial institution could use big data to identify high-net-worth individuals and tailor its advertising to their specific financial needs. These highly targeted campaigns deliver significantly higher click-through rates and conversion rates compared to traditional, broad-based advertising.
A/B Testing and Data-Driven Optimization
A/B testing, a cornerstone of data-driven marketing, involves comparing two versions of a marketing asset (e.g., an email subject line, a website landing page, or an ad creative) to determine which performs better. Big data significantly enhances A/B testing by providing the scale and speed necessary to analyze the results quickly and accurately. This allows marketers to rapidly iterate and optimize their campaigns, continuously improving their effectiveness. For instance, a company might A/B test different email subject lines to see which generates the highest open rates, or test different ad creatives to see which drives the most conversions. The data gathered from these tests informs future campaigns, leading to a continuous cycle of improvement and optimization.
Comparison of Traditional and Big Data-Driven Marketing
Traditional Method | Big Data Method |
---|---|
Broad, generalized targeting (e.g., mass mailings, television commercials) | Highly targeted campaigns based on detailed customer segmentation and profiling |
Limited ability to measure campaign effectiveness | Real-time monitoring and measurement of campaign performance using key metrics (e.g., click-through rates, conversion rates, ROI) |
Reliance on intuition and guesswork | Data-driven decision-making based on insights derived from large datasets |
Slow iteration and optimization cycles | Rapid iteration and optimization using A/B testing and other data-driven approaches |
Difficult to personalize customer interactions | Highly personalized customer experiences tailored to individual preferences and behaviors |