How business intelligence helps in decision making is no longer a question; it’s a fundamental shift in how organizations operate. In today’s data-driven world, businesses leverage the power of business intelligence (BI) to transform raw data into actionable insights, driving strategic advantages and operational efficiencies. This allows for proactive decision-making, moving beyond gut feelings and intuition to a data-backed approach that minimizes risk and maximizes opportunities.
From identifying emerging market trends to optimizing supply chain logistics, BI offers a comprehensive view of the business landscape. This allows for informed choices across all levels of an organization, from strategic planning to daily operations. Understanding how to effectively harness the power of BI is crucial for businesses aiming for sustainable growth and competitive dominance in the modern marketplace.
Understanding Business Intelligence (BI) in a Business Context: How Business Intelligence Helps In Decision Making
Business intelligence (BI) is the process of collecting, analyzing, and interpreting data to drive informed business decisions. It leverages technology and analytical methods to transform raw data into actionable insights, ultimately improving efficiency, profitability, and competitive advantage. A robust BI system is crucial for organizations of all sizes, providing a clear view of their performance and enabling proactive strategic planning.
Core Components of a Robust BI System
A successful BI system relies on several interconnected components working in harmony. These components ensure data is effectively collected, processed, analyzed, and visualized to support decision-making. A lack in any one area can significantly impact the overall effectiveness of the system. The key components include data warehousing, data mining, online analytical processing (OLAP), data visualization, and reporting. Data warehousing provides a centralized repository for structured and unstructured data from various sources. Data mining techniques extract patterns and trends from this data. OLAP allows for multi-dimensional analysis of data, facilitating quicker insights. Data visualization tools present this analyzed data in easily understandable charts and graphs. Finally, comprehensive reports summarize key findings and deliver actionable intelligence.
Types of BI Tools and Their Applications
Numerous BI tools cater to diverse business needs and scales. The choice of tool depends on factors such as budget, technical expertise, and specific analytical requirements. Relational Database Management Systems (RDBMS) like MySQL and PostgreSQL form the foundation for many BI systems, storing and managing the core data. Data visualization tools, such as Tableau and Power BI, excel at presenting complex data in an accessible format. These tools allow users to create interactive dashboards, charts, and reports that highlight key performance indicators (KPIs). Advanced analytics platforms, like SAS and SPSS, provide more sophisticated statistical modeling and predictive capabilities, enabling businesses to forecast future trends and optimize strategies. Finally, cloud-based BI solutions, such as Google BigQuery and Amazon Redshift, offer scalability and cost-effectiveness, particularly beneficial for rapidly growing businesses.
Examples of BI Transforming Raw Data into Actionable Insights
Consider a retail company tracking sales data. Raw data might consist of individual transactions, including product ID, purchase date, customer ID, and location. BI tools can analyze this data to identify best-selling products, popular purchase times, and customer demographics. This allows the retailer to optimize inventory, personalize marketing campaigns, and strategically locate new stores. Similarly, a manufacturing company can use BI to analyze production data, identifying bottlenecks in the manufacturing process. This information allows for process improvements, reduced production costs, and increased efficiency. In the healthcare sector, BI can analyze patient data to predict potential outbreaks of disease, enabling proactive interventions and improved public health outcomes.
Hypothetical BI System for a Small Startup Focusing on Customer Relationship Management (CRM)
For a small startup focusing on CRM, a cost-effective and user-friendly BI system is crucial. The system could leverage a cloud-based database like Google Cloud SQL to store customer data, including contact information, purchase history, and customer support interactions. A data visualization tool like Tableau could be integrated to create dashboards displaying key metrics such as customer acquisition cost, customer lifetime value, and customer churn rate. This system would allow the startup to track customer engagement, identify high-value customers, and personalize marketing efforts, leading to improved customer retention and revenue growth. The system could also incorporate simple predictive modeling to forecast future customer behavior based on past interactions, allowing the startup to proactively address potential issues and improve customer satisfaction.
BI’s Role in Strategic Decision-Making
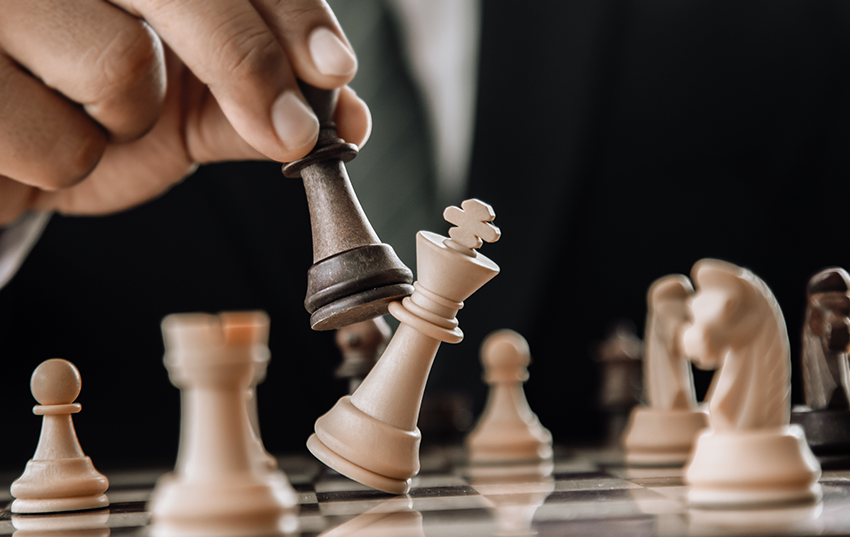
Business intelligence (BI) transcends simple data analysis; it empowers organizations to make informed, strategic decisions that drive growth and competitive advantage. By providing a comprehensive view of past performance, current trends, and future predictions, BI facilitates a shift from reactive firefighting to proactive strategic planning. This proactive approach allows businesses to anticipate challenges, capitalize on opportunities, and ultimately, achieve sustainable success.
BI facilitates proactive rather than reactive decision-making by providing timely and accurate insights into key performance indicators (KPIs). Instead of responding to crises as they arise, businesses can use BI to identify potential problems before they escalate, allowing for preventative measures and mitigating potential risks. This forward-looking approach enables strategic planning and resource allocation, maximizing efficiency and minimizing wasted effort.
Comparison of BI-Driven Decisions and Intuition-Based Decisions
BI-driven decisions are based on objective data analysis and statistical modeling, minimizing bias and emotional influences inherent in intuition-based decision-making. While gut feeling can sometimes prove surprisingly accurate, it lacks the rigorous validation and comprehensive context provided by BI. Intuition often relies on limited personal experience and may overlook critical data points. In contrast, BI leverages vast datasets, advanced analytics, and predictive modeling to provide a more holistic and reliable basis for strategic choices. The combination of data-driven insights and experienced judgment, however, often yields the best results.
Examples of Strategic Business Decisions Significantly Impacted by BI
Several high-profile companies have leveraged BI to make transformative strategic decisions. For instance, Netflix utilizes BI to analyze viewing habits, predict future trends in content preferences, and inform its substantial investments in original programming. This data-driven approach has been instrumental in Netflix’s global dominance in the streaming industry. Similarly, Amazon employs sophisticated BI systems to optimize its supply chain, personalize customer experiences, and dynamically adjust pricing strategies, contributing significantly to its e-commerce success. These examples highlight the transformative power of BI in driving strategic growth and competitive advantage.
Comparison of Different BI Approaches in Strategic Planning
BI Approach | Benefits | Drawbacks | Suitable for |
---|---|---|---|
Descriptive Analytics | Provides a clear understanding of past performance; identifies trends and patterns. | Limited predictive capabilities; doesn’t explain “why” trends occur. | Understanding historical performance; identifying areas for improvement. |
Predictive Analytics | Forecasts future outcomes; identifies potential risks and opportunities. | Requires large, high-quality datasets; model accuracy depends on data quality. | Risk management; resource allocation; strategic planning. |
Prescriptive Analytics | Recommends optimal actions; automates decision-making processes. | Implementation can be complex; requires significant technical expertise. | Supply chain optimization; pricing strategies; personalized marketing. |
Real-time Analytics | Provides immediate insights into ongoing operations; enables rapid response to changing conditions. | Requires robust infrastructure and data processing capabilities; data security concerns. | Customer service; fraud detection; operational efficiency monitoring. |
BI for Operational Efficiency and Improvement
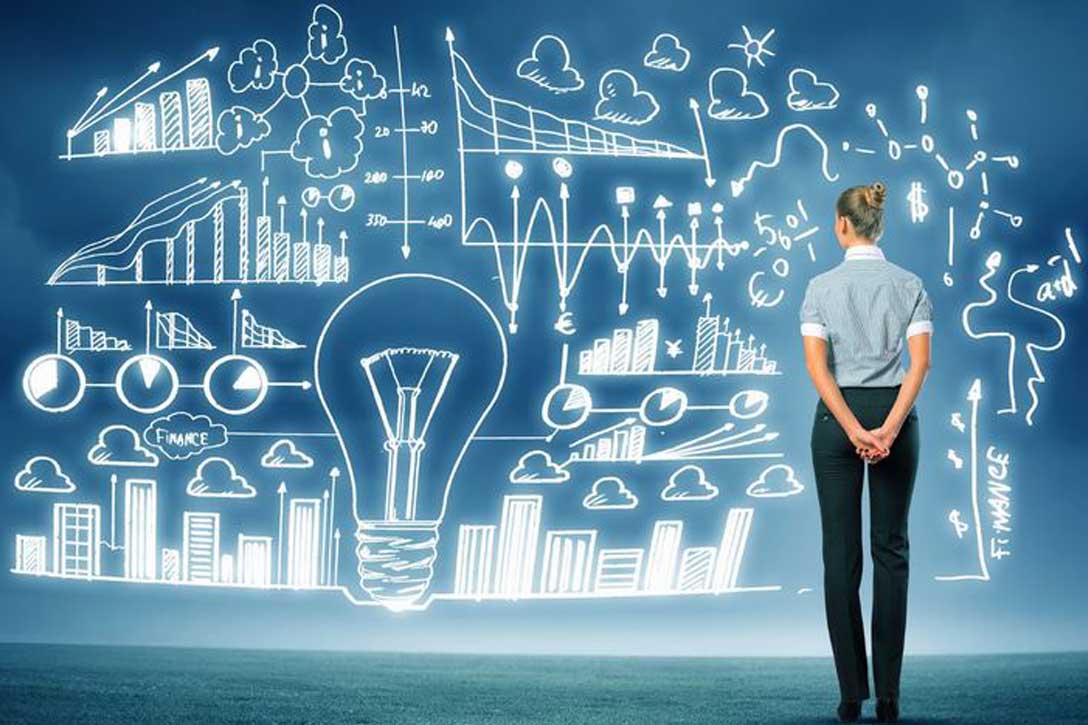
Business intelligence (BI) significantly enhances operational efficiency and fuels improvements across various business functions. By leveraging data analysis and visualization, organizations gain crucial insights into their operational processes, identifying bottlenecks, inefficiencies, and areas ripe for optimization. This leads to streamlined workflows, reduced operational costs, and ultimately, improved profitability.
BI’s impact on operational efficiency is multifaceted, impacting everything from inventory management to supply chain logistics. The ability to monitor key performance indicators (KPIs) in real-time allows for proactive adjustments, preventing potential problems before they escalate into costly disruptions. This proactive approach is a key differentiator between businesses that merely react to problems and those that actively anticipate and mitigate them.
Key Operational Areas Where BI Offers the Greatest Impact
BI offers substantial benefits across numerous operational areas. Its ability to analyze large datasets and identify trends provides actionable insights that lead to significant improvements. Specifically, areas like supply chain management, inventory control, customer service, and production processes see considerable gains from effective BI implementation. By identifying inefficiencies and optimizing resource allocation, BI empowers organizations to reduce operational costs and improve overall performance.
Streamlining Processes and Reducing Operational Costs with BI
BI streamlines processes by providing a clear, data-driven view of operational performance. This allows businesses to identify and eliminate redundant steps, automate manual tasks, and optimize resource allocation. For instance, analyzing sales data might reveal seasonal demand fluctuations, allowing businesses to adjust staffing levels accordingly, thus reducing labor costs. Similarly, identifying slow-moving inventory through BI analysis enables proactive strategies to reduce storage costs and prevent stock obsolescence. This proactive management of resources directly translates into significant cost savings.
Improving Supply Chain Management and Logistics with BI
Effective supply chain management hinges on accurate forecasting, timely delivery, and efficient inventory management. BI tools excel in providing the necessary insights to optimize these aspects. For example, by analyzing historical sales data, weather patterns, and economic indicators, BI can predict future demand, enabling businesses to proactively adjust their procurement strategies and avoid stockouts or overstocking. Real-time tracking of shipments using GPS data and integrated BI dashboards provides complete visibility into the supply chain, allowing for immediate responses to potential delays or disruptions. This proactive approach minimizes disruptions and improves overall supply chain efficiency.
Optimizing Inventory Management with BI: A Step-by-Step Process
Optimizing inventory management through BI involves a structured approach:
- Data Collection and Integration: Gather data from various sources, including sales transactions, purchase orders, and inventory levels. Integrate this data into a central BI system.
- KPI Definition and Monitoring: Define key inventory metrics such as inventory turnover rate, stockout rate, and carrying costs. Establish dashboards to monitor these KPIs in real-time.
- Demand Forecasting: Use historical sales data and predictive analytics to forecast future demand. Consider factors such as seasonality, promotions, and economic trends.
- Inventory Optimization: Based on demand forecasts and inventory levels, optimize stock levels to minimize carrying costs while avoiding stockouts. Employ techniques like ABC analysis to prioritize inventory management efforts.
- Real-time Monitoring and Adjustment: Continuously monitor inventory levels and adjust procurement strategies as needed. Use alerts to notify managers of potential stockouts or overstocking situations.
For example, a retailer using BI to analyze sales data might discover a consistent surge in demand for a particular product during the holiday season. This insight allows them to increase their inventory levels proactively, ensuring sufficient stock to meet the increased demand and avoid lost sales opportunities. Conversely, identifying slow-moving inventory allows for price adjustments or promotional strategies to clear out excess stock and reduce carrying costs.
BI and Data Visualization for Effective Communication
Data visualization is crucial for translating complex business intelligence findings into easily digestible information for stakeholders. Effective visuals transform raw data into compelling narratives, facilitating better understanding and driving informed decision-making. Without clear communication, even the most insightful BI analysis remains untapped potential.
Effective data visualization enhances understanding of complex data by simplifying intricate relationships and patterns. Instead of sifting through spreadsheets filled with numbers, stakeholders can quickly grasp key trends and outliers through visually appealing charts and graphs. This visual representation allows for quicker identification of critical issues, opportunities, and areas needing attention, ultimately leading to faster and more effective responses.
Chart and Graph Selection for BI Insights, How business intelligence helps in decision making
The choice of chart or graph significantly impacts the effectiveness of communication. Different visualization types are suited to different data types and analytical objectives. Selecting the appropriate visual aids is key to conveying insights accurately and efficiently.
- Line graphs: Ideal for showing trends over time, such as sales performance or website traffic.
- Bar charts: Effective for comparing different categories, such as sales figures across various product lines or customer demographics.
- Pie charts: Useful for illustrating proportions or percentages, such as market share or customer segmentation.
- Scatter plots: Excellent for identifying correlations between two variables, such as advertising spend and sales revenue.
- Heatmaps: Show data density across a matrix, useful for visualizing geographic data or identifying peak usage periods.
Communicating BI Findings to Stakeholders
Clear and concise communication of BI findings is paramount for successful implementation of data-driven strategies. This requires tailoring the presentation to the audience’s level of understanding and focusing on the key takeaways. The use of storytelling techniques, integrating visual aids with concise narratives, and highlighting actionable insights are vital for impactful communication. Avoid overwhelming stakeholders with excessive detail; instead, focus on the most critical information that directly influences decision-making.
Illustrative Example of BI-Driven Change Impact
Imagine a retail company using BI to analyze customer purchase data. The analysis reveals a significant drop in sales of a specific product line. A bar chart clearly displays the decline in sales over the past six months compared to the previous year. Using this data, the company implements a targeted marketing campaign focusing on highlighting the product’s unique features and offering a limited-time discount. A subsequent bar chart, presented alongside the initial one, showcases a significant increase in sales following the campaign. This visual comparison demonstrates the direct impact of the BI-driven change on the company’s bottom line, showing a clear upward trend in sales after the marketing intervention. The difference between the sales figures before and after the campaign is highlighted using a contrasting color, further emphasizing the positive impact of the data-driven decision. This visual representation provides a compelling narrative showcasing the ROI of the BI initiative.
BI and Risk Management

Business intelligence (BI) plays a crucial role in proactive risk management, transforming reactive approaches into predictive strategies. By leveraging data analysis and visualization, organizations can identify emerging risks, assess their potential impact, and implement mitigation plans, ultimately enhancing resilience and protecting their bottom line. This proactive approach not only reduces financial losses but also safeguards reputation and ensures business continuity.
BI facilitates risk identification and mitigation by providing a comprehensive view of the organization’s operational landscape. This holistic perspective, enabled by data aggregation and analysis, allows for the early detection of anomalies and potential threats that might otherwise go unnoticed. This early warning system is invaluable in preventing significant disruptions and losses.
Predictive Analytics in Risk Assessment
Predictive analytics, a key component of BI, leverages historical data and statistical modeling to forecast future events and assess the probability of various risks. For instance, by analyzing past sales data, market trends, and economic indicators, a company can predict potential declines in revenue and proactively adjust its pricing strategy or explore new market segments. Similarly, analyzing customer churn data can help predict customer attrition rates, allowing for targeted retention campaigns. This predictive capability moves risk management from a reactive to a proactive approach, enabling organizations to mitigate potential problems before they materialize. An example would be a telecommunications company using predictive modeling to identify customers at high risk of churning based on usage patterns and customer service interactions. This allows them to offer targeted promotions or improved customer service to retain these valuable customers.
BI and Fraud Detection
BI systems excel at detecting fraudulent activities by analyzing large datasets for anomalies and patterns indicative of fraud. For example, a bank might use BI to monitor transactions for unusual spending patterns, identifying potentially fraudulent credit card usage. This involves setting thresholds for acceptable transaction amounts, frequencies, and locations. Deviations from these norms trigger alerts, allowing for prompt investigation and prevention of further losses. Another example could be an e-commerce company using BI to detect fake reviews or fraudulent account creation attempts by analyzing user behavior, IP addresses, and review patterns. BI’s ability to process and analyze vast quantities of data in real-time makes it an invaluable tool in combating fraud.
Framework for Integrating BI into Risk Management
A robust framework for integrating BI into a company’s risk management strategy involves several key steps. First, identify key risk areas relevant to the business. This might include financial risks, operational risks, compliance risks, and reputational risks. Second, define key performance indicators (KPIs) to measure these risks. This could involve tracking metrics like late payments, production defects, regulatory fines, or negative social media sentiment. Third, implement BI tools and technologies capable of collecting, processing, and analyzing the relevant data. This might involve data warehousing, ETL processes, and advanced analytics platforms. Fourth, develop dashboards and reports to visualize the risk landscape and track KPIs. These visualizations should provide clear and concise insights into the organization’s risk profile. Finally, establish a process for responding to identified risks, including developing mitigation strategies and contingency plans. This requires a collaborative effort across different departments, ensuring effective communication and coordination. Regular review and refinement of this framework are essential to ensure its ongoing effectiveness in addressing evolving business risks.
Challenges and Limitations of Using BI in Decision-Making
Business intelligence (BI) offers powerful tools for data-driven decision-making, but its effective implementation and utilization are not without challenges. Overcoming these hurdles is crucial to realizing the full potential of BI and avoiding potentially costly mistakes. A thorough understanding of these limitations is essential for successful BI deployment and accurate interpretation of results.
Data Quality and Accuracy Issues
The reliability of BI-driven decisions hinges entirely on the quality of the underlying data. Inaccurate, incomplete, or inconsistent data will inevitably lead to flawed analyses and misguided strategies. Data quality problems can stem from various sources, including data entry errors, outdated information, inconsistent data formats across different systems, and a lack of proper data governance. For example, a retail company relying on BI for inventory management might make inaccurate sales forecasts if its sales data includes significant numbers of duplicate entries or missing information on returned goods. This could lead to overstocking or stockouts, impacting profitability. Robust data cleansing, validation, and ongoing monitoring are vital to mitigate these risks.
Misleading Conclusions from BI Analyses
While BI tools can process vast amounts of data, they cannot inherently interpret the meaning or context behind the data. Overreliance on BI visualizations without sufficient domain expertise can lead to misinterpretations and flawed conclusions. For instance, a correlation between two variables shown in a BI dashboard might be mistakenly interpreted as causation. A company might observe a correlation between increased advertising spending and higher sales, but this doesn’t automatically mean advertising directly caused the sales increase. Other factors could be at play, such as seasonal changes or competitor actions. It’s crucial to combine BI insights with expert judgment and contextual understanding to avoid drawing inaccurate or misleading conclusions.
Ethical Considerations in BI Applications
The use of BI raises several ethical concerns, particularly regarding data privacy, security, and bias. The collection and analysis of sensitive customer data necessitate stringent adherence to data privacy regulations (like GDPR or CCPA). Furthermore, biased algorithms or datasets used in BI systems can perpetuate and even amplify existing societal biases, leading to unfair or discriminatory outcomes. For example, a loan application system powered by BI might unfairly reject applications from individuals in specific demographic groups if the underlying data reflects historical biases in lending practices. Ethical BI implementation requires careful consideration of data security, privacy, and fairness, along with robust mechanisms for identifying and mitigating bias.
Implementation and Integration Challenges
Implementing a comprehensive BI system can be a complex and costly undertaking. Challenges include integrating data from disparate sources, ensuring data consistency, selecting appropriate BI tools and technologies, and training personnel to effectively use and interpret the generated insights. Furthermore, organizational resistance to change and a lack of buy-in from key stakeholders can hinder the successful adoption of a BI system. A well-defined implementation plan, robust change management strategies, and ongoing support are crucial for overcoming these hurdles.