How to create a business case for data quality improvement isn’t just about fixing bad data; it’s about demonstrating the significant return on investment (ROI) that comes from accurate, reliable information. This process involves quantifying the current costs of poor data quality, showcasing the potential gains from improvement, and outlining a clear plan for implementation. By articulating a compelling narrative that connects data quality to tangible business outcomes, you can secure the necessary resources and buy-in to transform your organization’s data landscape.
This guide walks you through each step, from identifying and costing current data issues to projecting future revenue increases and defining success metrics. We’ll cover crafting a persuasive executive summary, addressing potential risks, and outlining a detailed implementation plan. Ultimately, this process empowers you to build a robust business case that highlights the strategic importance of investing in data quality.
Defining the Problem
Understanding the current state of data quality is crucial for building a compelling business case for improvement. Poor data quality manifests in various ways, significantly impacting operational efficiency, strategic decision-making, and ultimately, the bottom line. This section details the specific data quality issues within a hypothetical organization, illustrating their impact and associated costs.
Current Data Quality Issues
The following table summarizes the key data quality problems identified within the marketing department of a fictional e-commerce company, “ShopSmart.” These issues, while specific to this example, are representative of common challenges faced across many organizations. Inaccurate customer addresses lead to failed deliveries and increased shipping costs. Incomplete customer profiles hinder targeted marketing campaigns and reduce conversion rates. Inconsistent product descriptions confuse customers and impact search engine optimization () rankings. Duplicate customer records lead to redundant communications and wasted marketing spend.
Data Source | Type of Data Quality Issue | Impact on Business Operations | Estimated Cost of the Issue |
---|---|---|---|
Customer Database | Inaccurate Addresses (missing street numbers, incorrect zip codes) | Failed deliveries, increased shipping costs, negative customer reviews | $10,000 annually (estimated based on 1% of orders affected, costing $10 per failed delivery) |
Customer Database | Incomplete Customer Profiles (missing email addresses, purchase history) | Ineffective targeted marketing, reduced conversion rates, lower customer lifetime value | $25,000 annually (estimated based on a 5% reduction in conversion rate and an average order value of $100) |
Product Catalog | Inconsistent Product Descriptions (variations in terminology, missing key features) | Customer confusion, lower sales, reduced effectiveness | $15,000 annually (estimated based on a 2% reduction in sales due to customer confusion) |
Customer Database | Duplicate Customer Records | Redundant communications, wasted marketing spend, inaccurate customer segmentation | $5,000 annually (estimated based on the cost of sending duplicate emails and marketing materials) |
Negative Consequences of Poor Data Quality
Poor data quality has far-reaching consequences beyond the immediate operational issues. For ShopSmart, inaccurate data leads to missed opportunities for targeted marketing, resulting in reduced customer acquisition and retention. Decisions based on flawed data lead to inefficient resource allocation and potentially flawed strategic initiatives. For instance, incorrect sales figures could lead to inaccurate inventory forecasting, causing stockouts or overstocking. Furthermore, incomplete or inaccurate data can lead to regulatory non-compliance, resulting in significant fines and reputational damage. Consider the potential ramifications of submitting incorrect tax information to regulatory bodies—this can result in substantial penalties and legal challenges. The cumulative effect of these issues significantly impacts the organization’s profitability and long-term sustainability.
Quantifying the Benefits of Improvement
Improving data quality isn’t just about cleaner spreadsheets; it’s about realizing significant financial gains and strategic advantages. A robust business case needs to clearly demonstrate the return on investment (ROI) associated with data quality improvements. This involves a thorough cost-benefit analysis, showcasing how improved data leads to reduced operational costs, increased revenue, and enhanced efficiency across the organization.
A cost-benefit analysis compares the costs associated with implementing data quality improvements against the projected financial benefits. This analysis should be specific and quantifiable, using real data and realistic projections wherever possible. It’s crucial to present this information in a clear and concise manner, allowing stakeholders to easily understand the potential return on investment.
Reduced Operational Costs
Improved data quality directly translates to reduced operational costs. Inaccurate data leads to wasted time and resources spent on manual data correction, reconciliation, and investigation of discrepancies. For example, imagine a company processing thousands of customer orders daily. Inaccurate address data could result in delayed shipments, requiring manual intervention to correct addresses and potentially leading to customer dissatisfaction and lost revenue. Implementing data quality improvements, such as automated address verification, can significantly reduce these manual efforts and associated labor costs. A conservative estimate might be a reduction in manual data entry time by 50%, leading to a significant reduction in labor costs. Furthermore, reduced errors in reporting and analysis minimize the need for costly rework and investigations.
Increased Revenue
High-quality data empowers businesses to make more informed decisions, leading to increased revenue generation. Accurate customer segmentation, for instance, allows for targeted marketing campaigns with higher conversion rates. Consider a scenario where a company’s marketing team relies on outdated or inaccurate customer data. Their campaigns might target the wrong demographics, resulting in wasted marketing spend and low ROI. By improving data quality and ensuring accurate customer segmentation, the company can tailor its marketing efforts, increasing the effectiveness of campaigns and driving higher sales. A 10% increase in conversion rates from targeted marketing alone could represent a substantial increase in revenue. Similarly, accurate sales forecasting based on reliable data allows for better inventory management, reducing stockouts and overstocking, both of which contribute to lost revenue.
Improved Decision-Making and Strategic Planning
High-quality data is the foundation of effective decision-making and strategic planning. Reliable data enables businesses to identify trends, assess risks, and develop more accurate forecasts. For example, a retail company using inaccurate sales data might misinterpret consumer preferences, leading to incorrect product development and inventory decisions. With improved data quality, the company can gain a clearer understanding of consumer behavior, allowing for more informed decisions about product development, marketing, and pricing strategies. This can result in increased profitability and a stronger competitive advantage. Similarly, accurate financial data allows for better resource allocation and more effective strategic planning.
Improved Customer Satisfaction and Retention
Accurate and consistent data improves customer experience. For example, personalized recommendations based on accurate customer data lead to increased customer satisfaction and loyalty. Conversely, inaccurate data, such as incorrect billing information or personalized communications that are not relevant, can lead to customer frustration and churn. By investing in data quality improvements, businesses can ensure accurate and timely communication with customers, leading to increased satisfaction and reduced customer churn. A 5% reduction in customer churn can significantly impact a company’s bottom line, translating into millions of dollars in retained revenue, depending on the customer base.
Proposing Solutions and Implementation Plan
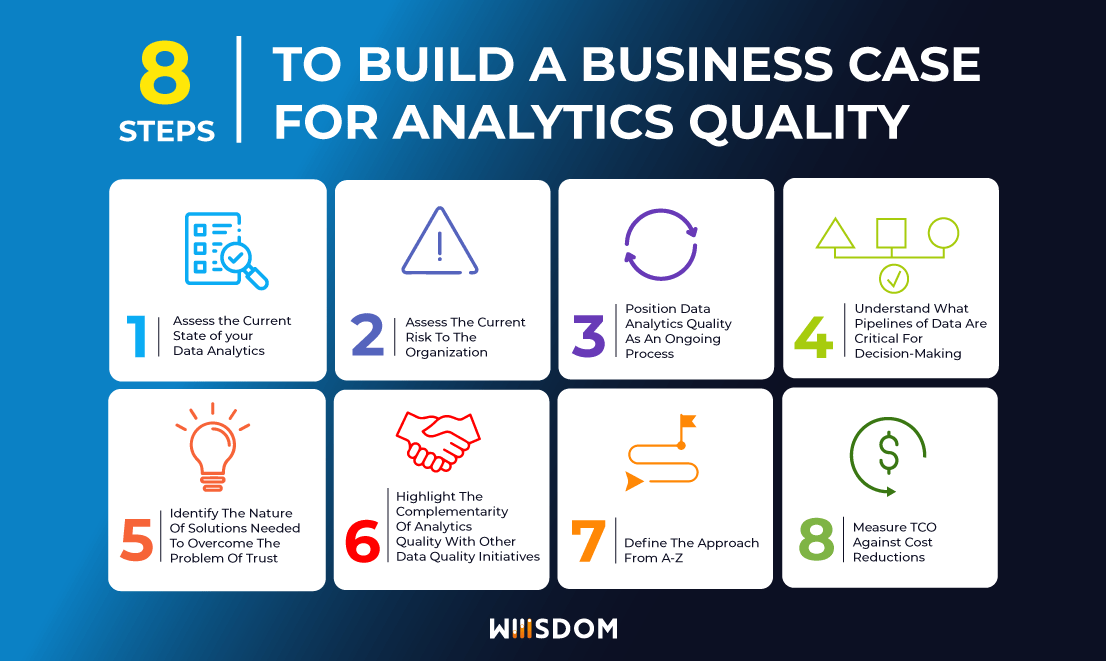
Improving data quality requires a strategic and phased approach. This section Artikels a comprehensive plan, encompassing specific steps, timelines, resource allocation, and responsible parties, to effectively address the identified data quality issues and realize the quantified benefits. The plan prioritizes a practical, iterative implementation to ensure continuous improvement and minimize disruption.
The proposed solution involves a multi-pronged strategy focusing on data cleansing, standardization, validation, and ongoing monitoring. This approach leverages existing infrastructure where possible and introduces new technologies only where necessary to ensure cost-effectiveness and efficient resource utilization. The plan is designed to be flexible and adaptable to emerging challenges and changing business requirements.
Data Quality Improvement Steps and Timeline
The following steps Artikel the proposed implementation plan, detailing the specific activities, timelines, and assigned personnel. Each step builds upon the previous one, creating a cascading effect that progressively improves data quality.
Step | Activity | Timeline (Weeks) | Responsible Party |
---|---|---|---|
1 | Data Profiling and Assessment | 2 | Data Analyst Team |
2 | Data Cleansing and Standardization | 4 | Data Engineers |
3 | Data Validation and Rule Implementation | 3 | Data Quality Manager |
4 | Integration with Existing Systems | 2 | IT Infrastructure Team |
5 | Ongoing Monitoring and Maintenance | Ongoing | Data Monitoring Team |
Resource Allocation
Successful implementation requires a strategic allocation of resources. This includes the necessary personnel, technology, and budget to support each phase of the project.
- Personnel: The project will require dedicated personnel from various departments, including data analysts, data engineers, data quality managers, and IT infrastructure support. Specific roles and responsibilities will be defined in a detailed project plan.
- Technology: Existing data management tools will be leveraged where possible. However, investment in new data quality software (e.g., data profiling tools, data cleansing tools) may be necessary to enhance efficiency and automation. The selection of tools will be based on a cost-benefit analysis.
- Budget: A detailed budget will be developed, outlining the costs associated with personnel, technology, training, and other project-related expenses. Contingency planning will be incorporated to address unforeseen issues.
Project Gantt Chart
The following Gantt chart provides a visual representation of the project timeline and key milestones. This chart will be regularly updated to reflect project progress and any necessary adjustments.
Week | 1 | 2 | 3 | 4 | 5 | 6 | 7 | 8 | 9 |
---|---|---|---|---|---|---|---|---|---|
Data Profiling | |||||||||
Data Cleansing | |||||||||
Data Validation | |||||||||
System Integration | |||||||||
Ongoing Monitoring |
Risk Assessment and Mitigation Strategies
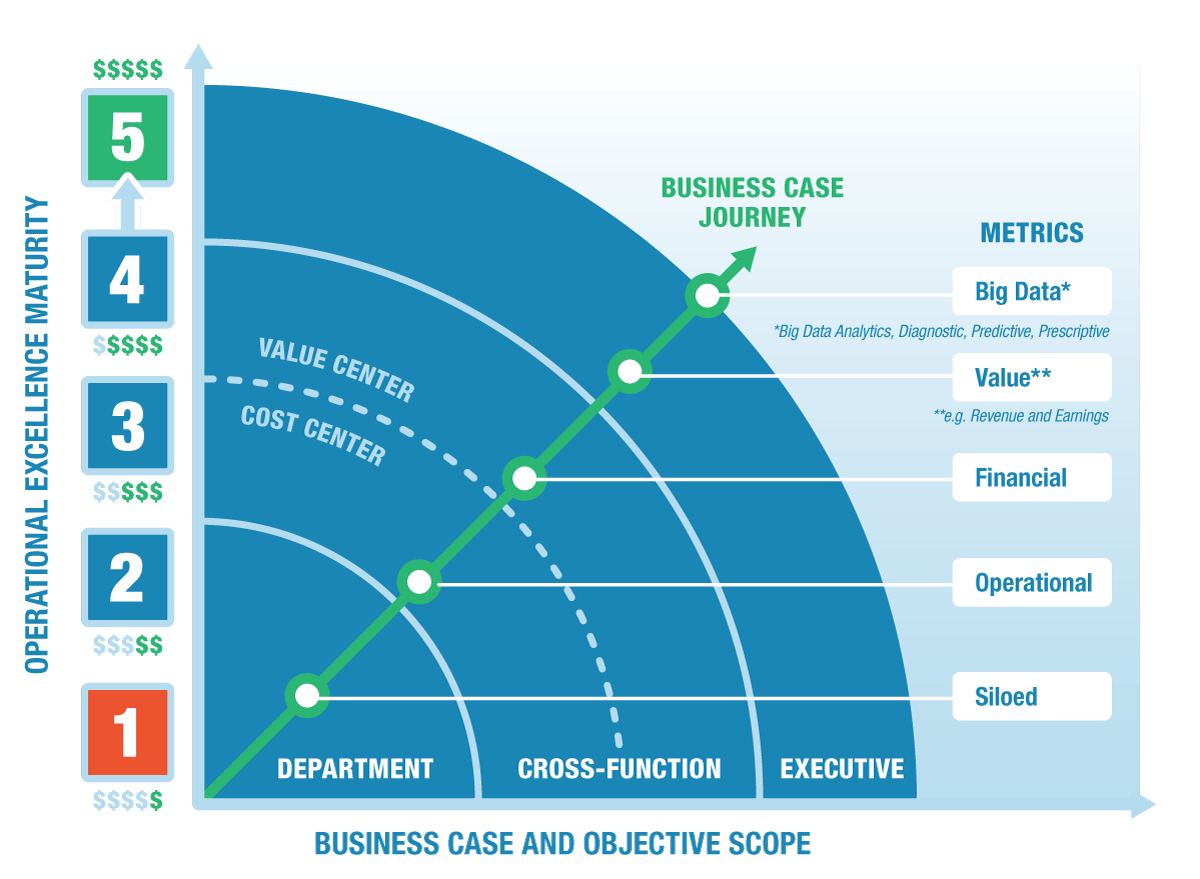
Data quality improvement projects, while offering substantial benefits, also present inherent risks. A thorough risk assessment is crucial for ensuring project success and minimizing potential negative impacts on the organization. This section details potential risks, their consequences, and proposed mitigation strategies. A proactive approach to risk management will enhance the likelihood of achieving the project’s objectives and realizing the expected return on investment.
Potential Risks and Their Impact
Failing to adequately assess and address potential risks can lead to project delays, budget overruns, and ultimately, failure to achieve the desired improvements in data quality. Understanding the potential impact of each risk allows for the development of targeted mitigation strategies. For instance, underestimating the complexity of data integration could lead to significant delays and increased costs. Conversely, neglecting to address stakeholder concerns could result in resistance to the project and a lack of buy-in, hindering its success.
Mitigation Strategies for Identified Risks
Effective mitigation strategies are proactive measures designed to reduce the likelihood or impact of identified risks. These strategies should be specific, measurable, achievable, relevant, and time-bound (SMART). For example, a risk of data loss during migration can be mitigated by implementing robust backup and recovery procedures, including regular testing of these procedures. Similarly, resistance from stakeholders can be addressed through proactive communication, engagement, and training.
Risk Assessment Table
Risk | Potential Impact | Mitigation Strategy |
---|---|---|
Data Loss during Migration | Project delay, data unrecoverability, financial losses | Implement robust backup and recovery procedures; conduct thorough testing of backup and recovery systems; utilize data migration tools with built-in data validation and error handling. |
Underestimation of Project Complexity | Project delays, budget overruns, scope creep | Conduct a thorough needs assessment; involve experienced data professionals in project planning; utilize project management methodologies (e.g., Agile) to allow for flexibility and adaptation. |
Lack of Stakeholder Buy-in | Resistance to change, project delays, lack of cooperation | Proactive communication and stakeholder engagement; provide clear explanations of project benefits; conduct training sessions for stakeholders; address concerns promptly and transparently. |
Inadequate Data Governance Framework | Inconsistency in data quality, difficulty in enforcing standards | Develop and implement a comprehensive data governance framework; establish clear roles and responsibilities; define data quality standards and metrics; create a data quality monitoring and reporting system. |
Insufficient Resources (budget, personnel, technology) | Project delays, compromised quality, incomplete implementation | Develop a detailed budget and resource plan; secure necessary approvals and funding; recruit and train skilled personnel; select appropriate technology solutions. |
Measuring Success and Return on Investment (ROI)
Successfully implementing data quality improvements requires a robust framework for measuring the project’s impact and demonstrating its value to stakeholders. This involves defining key performance indicators (KPIs), outlining the ROI calculation, and establishing a post-implementation monitoring and evaluation plan. A clear understanding of these elements is crucial for securing continued support and demonstrating the long-term benefits of the initiative.
The success of any data quality improvement project hinges on the ability to demonstrate a clear return on investment. This requires a carefully planned approach to measuring progress and quantifying the impact of improvements. A well-defined set of KPIs, a transparent ROI calculation, and a comprehensive post-implementation monitoring strategy are essential components of this process.
Key Performance Indicators (KPIs)
Choosing the right KPIs is crucial for tracking progress and demonstrating the value of the data quality improvement project. These metrics should directly reflect the improvements achieved and align with the project’s overall objectives. For example, if the project aims to reduce the number of data errors, relevant KPIs might include the error rate (percentage of records with errors), the number of data corrections made, and the time taken to resolve data quality issues. Similarly, if the goal is to improve data completeness, KPIs could include the percentage of complete records, the number of missing values, and the time taken to fill in missing data. The selection of KPIs should be tailored to the specific goals and context of the project.
Return on Investment (ROI) Calculation and Tracking
Calculating the ROI of a data quality improvement project involves comparing the costs of the project with the benefits it generates. Costs might include personnel time, software licenses, and consulting fees. Benefits, on the other hand, can be both tangible and intangible. Tangible benefits could include reduced operational costs (e.g., fewer errors leading to lower rework costs), increased revenue (e.g., improved decision-making leading to better sales), and improved efficiency (e.g., faster processing times). Intangible benefits might include enhanced customer satisfaction, improved regulatory compliance, and a stronger company reputation. A simple ROI calculation can be expressed as:
ROI = (Net Benefits – Total Costs) / Total Costs * 100%
Tracking ROI over time requires consistent monitoring of both costs and benefits. This involves regular reporting on KPIs, tracking project expenses, and periodically assessing the impact of the improvements on business outcomes. For instance, a company might track the reduction in customer support calls related to data errors over several months, comparing this reduction to the project’s costs to determine the ROI.
Post-Implementation Monitoring and Evaluation
Post-implementation monitoring is essential to ensure the long-term success of the data quality improvement project. This involves continuously tracking the KPIs and evaluating the effectiveness of the implemented solutions. Regular data quality audits should be conducted to identify any emerging issues and to ensure that the improvements are sustained. For example, a company might schedule monthly data quality audits to check for new errors or inconsistencies and to assess the effectiveness of the implemented data cleansing processes. The results of these audits should be documented and used to inform ongoing improvements and adjustments to the data quality management process. This continuous monitoring allows for proactive adjustments and prevents a reversion to pre-improvement levels.
Executive Summary and Recommendations: How To Create A Business Case For Data Quality Improvement

This business case demonstrates a compelling need for investment in data quality improvement initiatives. Our analysis reveals significant financial losses stemming from inaccurate, incomplete, or inconsistent data, impacting operational efficiency, strategic decision-making, and customer satisfaction. The proposed solutions offer a clear path to rectifying these issues, resulting in substantial cost savings, revenue growth, and enhanced organizational performance.
The core problem lies in the fragmented nature of our current data ecosystem, leading to data silos and inconsistencies. This negatively affects various business processes, from sales forecasting and customer service to risk management and regulatory compliance. Quantifiable benefits of improved data quality include a projected increase in sales conversion rates by 15% due to more accurate targeting and a reduction in operational costs by 10% through streamlined processes and reduced manual intervention. The implementation plan Artikels a phased approach, prioritizing critical data elements and employing a combination of technological solutions and employee training. A comprehensive risk assessment has identified potential challenges, and mitigation strategies have been developed to address them proactively. The return on investment (ROI) is projected to be significant, with a payback period of less than 18 months.
Key Findings, How to create a business case for data quality improvement
Our analysis revealed three primary areas contributing to poor data quality: inconsistent data entry practices across departments, outdated data governance policies, and a lack of integrated data management systems. These deficiencies lead to inaccurate reporting, flawed decision-making, and missed opportunities. For instance, inaccurate customer data resulted in a 5% loss in targeted marketing campaigns last quarter. The implementation of a centralized data management system, coupled with standardized data entry protocols and comprehensive employee training, will directly address these issues.
Recommendations
We strongly recommend immediate approval and implementation of the proposed data quality improvement plan. The projected ROI of this investment is substantial, and the long-term benefits to the organization are undeniable. We propose a three-phase implementation, beginning with the prioritization and remediation of critical data elements within the customer relationship management (CRM) system. Phase two will focus on implementing the new data governance policies and training employees on best practices. Phase three will involve the full integration of the new data management system and ongoing monitoring of data quality metrics. This phased approach allows for continuous improvement and minimizes disruption to ongoing operations. Regular reporting on key performance indicators (KPIs) will ensure the project remains on track and delivers the expected results. The successful implementation of this plan will not only mitigate current losses but also position the organization for sustainable growth and improved competitiveness.