Is business analytics STEM? Absolutely. This field blends the rigorous quantitative methods of science, technology, engineering, and mathematics (STEM) with the strategic acumen needed to solve real-world business challenges. From predicting customer behavior to optimizing supply chains, business analytics leverages data-driven insights to inform critical decision-making across diverse industries. This exploration delves into the core components of business analytics, its relationship to STEM disciplines, essential skills, lucrative career paths, and the exciting future it holds.
We’ll examine the specific STEM skills—like statistical modeling, programming, and data visualization—that are crucial for success in business analytics. We will also cover the educational routes that seamlessly integrate STEM knowledge with business principles, preparing professionals for impactful careers at the intersection of data and decision-making. The analysis will extend to include a comparison with data science, highlighting the unique skill sets and career trajectories within each field.
Defining Business Analytics
Business analytics is the process of transforming raw data into actionable insights that inform strategic decision-making within an organization. It leverages statistical methods, data mining techniques, and visualization tools to uncover patterns, trends, and anomalies in data, ultimately driving improvements in efficiency, profitability, and competitive advantage. This discipline goes beyond simple reporting; it actively seeks to understand *why* things are happening, not just *what* is happening.
Core Components of Business Analytics
Business analytics relies on a synergistic interplay of several key components. Data collection forms the foundation, gathering relevant information from various sources, both internal and external. Data warehousing and management then organize and cleanse this raw data, making it suitable for analysis. Data mining techniques, such as regression analysis and clustering, are employed to uncover hidden relationships and patterns. Finally, data visualization tools present these findings in a clear and concise manner, facilitating easy interpretation and communication of insights to stakeholders. The entire process is iterative, with insights leading to further data collection and analysis in a continuous feedback loop.
Applications of Business Analytics Across Industries
The applications of business analytics are incredibly diverse, spanning numerous industries. In retail, it helps optimize pricing strategies, predict demand, and personalize customer experiences. Financial institutions use it for fraud detection, risk management, and algorithmic trading. Manufacturing benefits from predictive maintenance, optimizing production processes, and managing supply chains. Healthcare utilizes business analytics for improving patient outcomes, managing resources effectively, and identifying disease outbreaks. Even the public sector employs it for resource allocation, urban planning, and improving public services. The common thread is the use of data-driven insights to improve operational efficiency and strategic decision-making.
Examples of Business Analytics Solving Real-World Business Problems
Consider a major online retailer using business analytics to personalize product recommendations. By analyzing past purchase history, browsing behavior, and demographic data, the retailer can offer highly relevant product suggestions, increasing sales conversion rates significantly. Another example is a telecommunications company using predictive modeling to identify customers at high risk of churn. By analyzing call records, usage patterns, and customer service interactions, the company can proactively intervene with targeted retention offers, reducing customer churn and maintaining profitability. A manufacturing company might employ predictive maintenance to anticipate equipment failures. By analyzing sensor data from machinery, the company can schedule maintenance proactively, minimizing downtime and reducing repair costs.
Comparison of Descriptive, Predictive, and Prescriptive Analytics
Type of Analytics | Description | Example |
---|---|---|
Descriptive Analytics | Summarizes historical data to understand past performance. | Analyzing sales figures from the past year to identify top-selling products. |
Predictive Analytics | Uses historical data to forecast future outcomes. | Predicting customer churn based on past behavior and demographics. |
Prescriptive Analytics | Recommends actions to optimize future outcomes. | Suggesting optimal pricing strategies to maximize revenue based on predicted demand. |
STEM Fields and Their Relation to Business Analytics: Is Business Analytics Stem
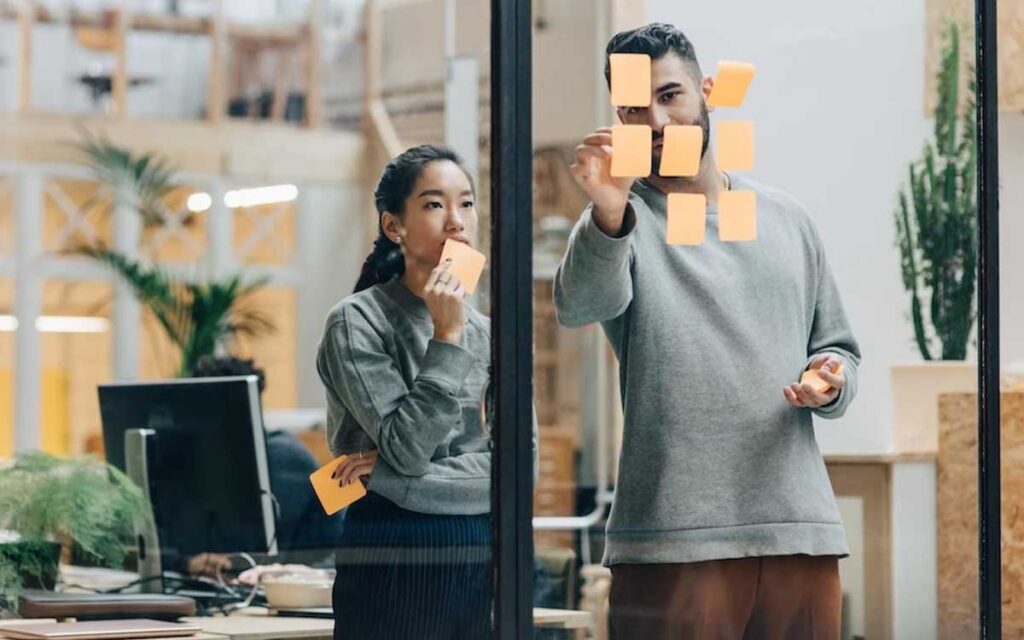
Business analytics, a field focused on extracting actionable insights from data to drive better business decisions, draws heavily upon the principles and methodologies of several STEM (Science, Technology, Engineering, and Mathematics) disciplines. The quantitative nature of the work, coupled with the need for sophisticated analytical techniques, makes a strong STEM foundation invaluable for success in this rapidly growing field.
The synergy between STEM and business analytics is evident in the core skills required. Professionals in this field need to be comfortable working with large datasets, building predictive models, and communicating complex findings to non-technical audiences. These abilities are directly related to the knowledge and skills developed within various STEM fields.
Relevant STEM Disciplines in Business Analytics
The most relevant STEM disciplines contributing to business analytics are mathematics, statistics, computer science, and engineering. These fields provide the foundational knowledge and technical expertise necessary to effectively analyze data and develop data-driven solutions.
Each of these fields contributes unique skills and perspectives to the field of business analytics. A solid understanding of these contributions is essential for anyone seeking a career in this dynamic area.
Mathematical and Statistical Skills in Business Analytics
Mathematics and statistics form the bedrock of business analytics. Mathematical skills, such as algebra, calculus, and linear algebra, are crucial for understanding and implementing various analytical techniques. Statistical knowledge, including probability theory, hypothesis testing, and regression analysis, is essential for drawing valid inferences from data and building predictive models. For example, understanding regression models allows analysts to predict sales based on marketing spend or customer demographics. Furthermore, proficiency in statistical software packages like R or Python is vital for practical application of these statistical methods.
Computer Science Skills in Business Analytics
Computer science skills are increasingly important in business analytics due to the ever-increasing volume and complexity of data. Proficiency in programming languages like Python, R, SQL, and Java is essential for data manipulation, cleaning, and analysis. Furthermore, knowledge of database management systems (DBMS) is crucial for efficient data storage and retrieval. A deep understanding of algorithms and data structures is also valuable for optimizing analytical processes and building efficient models. For instance, knowledge of SQL allows analysts to efficiently query and extract relevant data from large databases, while proficiency in Python enables the development of custom data analysis pipelines and machine learning models.
Engineering Skills in Business Analytics
While less directly involved than mathematics and computer science, engineering principles contribute significantly to business analytics. The problem-solving and analytical skills honed in engineering disciplines are directly transferable. Engineers are often trained in systematic approaches to problem-solving, data analysis, and model building, skills that are highly valuable in extracting meaningful insights from complex datasets. Furthermore, an understanding of systems thinking, a key concept in engineering, helps analysts understand the interdependencies within complex business processes and develop more comprehensive solutions. For example, an engineer’s understanding of process optimization can be applied to improve the efficiency of a supply chain.
Educational Pathways Combining STEM and Business Analytics
Numerous educational pathways combine STEM and business analytics. Many universities offer undergraduate and graduate degrees specifically in business analytics, often incorporating significant components from mathematics, statistics, and computer science. Other pathways involve pursuing a STEM undergraduate degree (e.g., mathematics, statistics, computer science, or engineering) followed by a master’s degree in business analytics or a related field. This dual approach provides a strong foundation in both quantitative skills and business acumen. Furthermore, numerous online courses and boot camps provide focused training in specific aspects of business analytics, allowing professionals from diverse backgrounds to upskill or transition into the field.
Successful Careers Bridging STEM and Business Analytics
Successful careers bridging STEM and business analytics are diverse and plentiful. Examples include data scientists, who leverage their STEM knowledge to build predictive models and extract insights from data; business intelligence analysts, who use data to improve business processes and decision-making; and machine learning engineers, who develop and deploy machine learning algorithms to solve business problems. These roles often involve collaborating with other business professionals, requiring strong communication and interpersonal skills in addition to technical expertise. The increasing demand for data-driven decision-making across various industries ensures a continued high demand for professionals with this unique combination of skills.
Required Skills and Knowledge
A successful career in business analytics requires a blend of technical proficiency, analytical thinking, and strong interpersonal skills. Individuals must be adept at manipulating data, interpreting results, and communicating findings effectively to both technical and non-technical audiences. This section details the essential skills and knowledge needed to thrive in this dynamic field.
Essential Technical Skills
Technical skills form the bedrock of any business analytics role. Proficiency in these areas allows analysts to effectively collect, clean, analyze, and visualize data to drive informed business decisions. A strong foundation in these areas is crucial for success.
- Programming Languages: Python and R are dominant languages in business analytics, offering extensive libraries for data manipulation, statistical analysis, and machine learning. SQL is essential for database management and querying.
- Data Wrangling and Preprocessing: This involves cleaning, transforming, and preparing raw data for analysis. Skills in handling missing values, outliers, and inconsistent data formats are critical.
- Statistical Analysis: A solid understanding of statistical methods, including hypothesis testing, regression analysis, and time series analysis, is crucial for drawing meaningful insights from data.
- Data Visualization: The ability to create clear and compelling visualizations using tools like Tableau, Power BI, or Python libraries (Matplotlib, Seaborn) is vital for communicating analytical findings effectively.
- Machine Learning Techniques: While not always required at an entry level, familiarity with machine learning algorithms (e.g., regression, classification, clustering) is increasingly valuable for advanced analytics roles.
Essential Soft Skills
While technical skills are foundational, soft skills are equally important for success in business analytics. These skills enable effective collaboration, communication, and problem-solving within a business context.
- Communication: The ability to clearly and concisely communicate complex analytical findings to both technical and non-technical audiences is paramount. This includes written reports, presentations, and verbal explanations.
- Problem-Solving: Business analytics often involves tackling ambiguous problems. Strong analytical and critical thinking skills are needed to define problems, develop solutions, and evaluate results.
- Collaboration: Business analysts frequently work in teams with individuals from various departments. Effective collaboration and teamwork are crucial for successful project completion.
- Business Acumen: Understanding business principles, processes, and strategies is essential for applying analytical insights to real-world business problems. This includes knowledge of finance, marketing, and operations.
- Critical Thinking: The ability to evaluate information objectively, identify biases, and draw sound conclusions from data is essential for producing reliable and actionable insights.
Hypothetical Business Analytics Program Curriculum
A comprehensive business analytics program should integrate STEM components throughout the curriculum. This example highlights key areas.
Course | STEM Component | Description |
---|---|---|
Calculus I & II | Mathematics | Foundation in mathematical concepts crucial for statistical modeling and machine learning. |
Linear Algebra | Mathematics | Essential for understanding matrix operations and algorithms used in data analysis. |
Probability and Statistics | Mathematics/Statistics | Fundamental understanding of probability distributions, hypothesis testing, and statistical inference. |
Data Structures and Algorithms | Computer Science | Essential for efficient data manipulation and algorithm design in large datasets. |
Database Management Systems | Computer Science | Understanding relational databases, SQL, and data warehousing techniques. |
Programming for Data Analysis (Python/R) | Computer Science | Practical application of programming languages for data manipulation, analysis, and visualization. |
Machine Learning | Computer Science/Statistics | Introduction to various machine learning algorithms and their applications in business. |
Data Visualization and Communication | Computer Science/Communication | Techniques for creating effective visualizations and communicating analytical findings. |
Business Strategy and Analytics | Business | Application of analytical techniques to solve real-world business problems. |
Data Science vs. Business Analytics: Skill Comparison
While both fields involve data analysis, their focuses and required skills differ. Data science often emphasizes developing new algorithms and models, requiring deeper expertise in advanced statistical modeling and machine learning. Business analytics focuses on applying existing techniques to solve specific business problems, requiring stronger business acumen and communication skills. Data scientists might build predictive models for fraud detection, while business analysts might use those models to optimize marketing campaigns.
The Future of Business Analytics
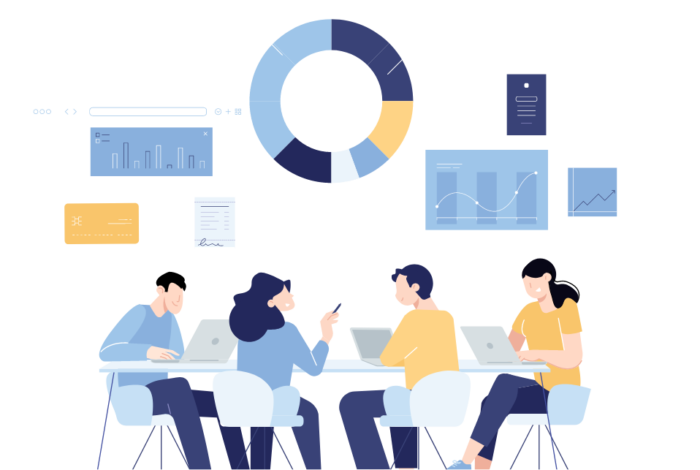
Business analytics is rapidly evolving, driven by technological advancements and the increasing reliance of organizations on data-driven decision-making. The future of this field promises both immense opportunities and significant challenges, requiring professionals to adapt and evolve their skillsets to remain competitive. This section explores key trends, predictions, and ethical considerations shaping the future landscape of business analytics.
Emerging Trends and Technologies
Several emerging trends and technologies are significantly impacting the field of business analytics. The integration of artificial intelligence (AI), particularly machine learning (ML) and deep learning (DL), is revolutionizing data analysis, enabling more accurate predictions, automated insights, and the identification of complex patterns previously undetectable by traditional methods. For example, AI-powered predictive models are increasingly used in fraud detection, customer churn prediction, and supply chain optimization, significantly improving efficiency and profitability. Furthermore, the rise of big data and the development of advanced analytics platforms capable of processing and analyzing massive datasets are unlocking new opportunities for extracting valuable insights. The increasing adoption of cloud computing provides scalable and cost-effective solutions for storing and processing these vast amounts of data. Finally, the growth of the Internet of Things (IoT) is generating an unprecedented volume of data from connected devices, creating new avenues for business analytics to extract meaningful insights from diverse sources.
Predictions about Future Demand for Business Analytics Professionals
The demand for skilled business analytics professionals is expected to continue its upward trajectory. The increasing reliance on data-driven decision-making across all industries, coupled with the complexity of analyzing large and diverse datasets, necessitates a skilled workforce capable of extracting actionable insights. According to a recent report by Gartner, the demand for data scientists and business analysts is projected to grow by 25% annually for the next five years. This growth is fueled by the need for organizations to leverage data to improve operational efficiency, gain a competitive advantage, and personalize customer experiences. For instance, the healthcare industry is experiencing a surge in demand for analytics professionals to analyze patient data and improve healthcare outcomes. Similarly, the financial services sector requires professionals to detect fraud, manage risk, and personalize financial products.
Ethical Considerations Related to Business Analytics
The increasing power and pervasiveness of business analytics raise significant ethical considerations. Bias in algorithms, data privacy concerns, and the potential for misuse of insights are crucial areas that require careful attention. Algorithmic bias, stemming from biased data used to train models, can perpetuate and amplify existing societal inequalities. For example, a loan application algorithm trained on historical data reflecting discriminatory lending practices may continue to discriminate against certain demographic groups. Protecting the privacy of individuals whose data is used in analytics is paramount. Regulations like GDPR in Europe and CCPA in California highlight the growing importance of data privacy and the need for transparent and responsible data handling practices. Finally, the potential misuse of analytics insights, such as for manipulative marketing or discriminatory practices, necessitates the establishment of clear ethical guidelines and regulatory frameworks.
Hypothetical Scenario: Business Analytics in a Future Business Environment
Imagine a future smart city utilizing a comprehensive network of interconnected sensors and IoT devices. A business analytics team within the city’s transportation department utilizes AI-powered predictive models to analyze real-time traffic data, weather patterns, and public transportation usage. These models forecast potential traffic congestion hotspots, allowing the city to dynamically adjust traffic light timings, optimize public transport routes, and proactively inform commuters of potential delays through a personalized mobile app. This data-driven approach leads to reduced traffic congestion, improved commute times, reduced fuel consumption, and enhanced overall quality of life for city residents. The analytics team also monitors citizen feedback gathered through the app, integrating this qualitative data with quantitative data to continuously improve the city’s transportation system. This scenario highlights how advanced business analytics, powered by AI and IoT, can contribute to solving complex urban challenges and improving the efficiency and effectiveness of public services.
Illustrative Examples

The power of business analytics is best understood through real-world applications. Examining successful case studies reveals how data-driven insights translate into tangible business improvements, impacting profitability, efficiency, and competitive advantage. The following examples illustrate the transformative potential of business analytics across diverse industries.
Netflix’s Use of Business Analytics for Content Recommendation and Personalization
Netflix’s success is heavily reliant on its sophisticated recommendation engine. This system analyzes vast amounts of user data – viewing history, ratings, search queries, and even the time spent watching specific scenes – to predict what each individual user might enjoy. This goes beyond simple genre recommendations; it considers nuances in taste, identifying patterns and correlations that humans might miss. For example, if a user consistently watches documentaries about nature and also enjoys historical dramas, the algorithm might suggest documentaries focusing on historical natural events. This personalized approach significantly increases user engagement, leading to higher retention rates and reduced churn. The effectiveness of this system is measurable through key performance indicators (KPIs) like average viewing time per user and subscriber retention. The data-driven approach allows Netflix to continually refine its algorithms, optimizing content recommendations and maximizing user satisfaction. This continuous improvement cycle is a hallmark of successful business analytics implementation.
A Retail Company Optimizing Inventory Management Through Predictive Analytics, Is business analytics stem
A large retail chain faced significant challenges with inventory management. Overstocking resulted in high storage costs and potential losses due to outdated products, while understocking led to lost sales and dissatisfied customers. They implemented a predictive analytics project using historical sales data, weather patterns, and economic indicators to forecast demand more accurately. The model considered seasonality, promotional periods, and even local events that might influence consumer behavior. The result was a significant reduction in inventory holding costs and a substantial increase in sales. Specifically, the company reduced its inventory holding costs by 15% and increased sales by 8% within the first year of implementing the predictive analytics system. This was achieved by optimizing stock levels in each store based on the predictions generated by the model, ensuring that popular items were always available and less popular items were not overstocked. This project demonstrated the ability of business analytics to optimize supply chain management and improve profitability.