Which of these are examples of business analytics? This question unveils the power of data-driven decision-making. Business analytics isn’t just about crunching numbers; it’s about transforming raw data into actionable insights that propel businesses forward. We’ll explore various techniques, from descriptive analysis revealing past trends to predictive modeling forecasting future outcomes, and even prescriptive analytics guiding optimal strategies. This journey will illuminate how businesses across diverse sectors leverage these powerful tools to gain a competitive edge and achieve strategic goals.
Understanding business analytics requires differentiating it from related fields like data science or business intelligence. While overlapping, business analytics focuses specifically on applying data analysis techniques to solve business problems and improve decision-making. This involves identifying key performance indicators (KPIs), analyzing trends, and developing strategies to optimize processes and achieve business objectives. The core is using data to inform strategic choices, leading to enhanced profitability, efficiency, and competitive advantage.
Defining Business Analytics
Business analytics is the process of discovering, interpreting, and communicating meaningful patterns in data to drive informed business decisions. It leverages various statistical methods, data mining techniques, and visualization tools to transform raw data into actionable insights, differentiating itself from data science, which focuses more on building predictive models and algorithms, and data visualization, which primarily concentrates on the presentation of data. While overlapping in some areas, business analytics is specifically geared towards solving business problems and improving organizational performance.
Business analytics aims to provide a competitive edge by enhancing decision-making across all aspects of an organization. Core objectives include improving operational efficiency, optimizing resource allocation, identifying new revenue streams, mitigating risks, and understanding customer behavior. Effective business analytics directly contributes to achieving strategic goals by providing data-driven evidence to support choices, ultimately leading to better outcomes and a higher return on investment.
Characteristics of Effective Business Analytics Practices
Effective business analytics is characterized by several key attributes. First, it relies on a robust data infrastructure capable of collecting, storing, and processing large volumes of data from various sources. Second, it employs a rigorous and methodical approach to data analysis, utilizing appropriate statistical techniques and ensuring the accuracy and reliability of findings. Third, effective business analytics emphasizes clear communication of insights through compelling visualizations and easily understandable reports tailored to the specific audience and their decision-making needs. Finally, successful business analytics initiatives are iterative and adaptive, constantly refining methodologies and models based on feedback and evolving business needs. For instance, a retail company might use business analytics to analyze sales data to identify seasonal trends, predict future demand, and optimize inventory management, leading to reduced costs and increased profits. This continuous improvement cycle is critical for ensuring that the insights derived from business analytics remain relevant and valuable over time.
Types of Business Analytics Techniques
Business analytics employs a range of techniques to extract insights from data, ultimately driving better decision-making. These techniques can be broadly categorized into descriptive, predictive, and prescriptive analytics, each offering unique capabilities and serving different analytical purposes. Understanding these distinctions is crucial for selecting the appropriate method for a specific business problem.
Descriptive analytics forms the foundation, providing a summary of past performance. Predictive analytics moves beyond this, forecasting future outcomes. Finally, prescriptive analytics goes a step further, recommending actions to optimize results. The choice of technique depends on the nature of the business question and the available data.
Descriptive Analytics Techniques
Descriptive analytics focuses on summarizing historical data to understand what has happened. Common techniques include data aggregation, data mining, and data visualization. These methods help identify trends, patterns, and outliers in existing data, providing a clear picture of past performance. For example, a retail company might use descriptive analytics to analyze sales data to identify the best-selling products in a particular region over the past quarter. This information can then inform inventory management and marketing strategies.
Predictive Analytics Techniques
Predictive analytics uses historical data and statistical algorithms to forecast future outcomes. Techniques like regression analysis, time series analysis, and machine learning algorithms are frequently employed. For instance, a bank might use predictive analytics to assess the creditworthiness of loan applicants, minimizing the risk of defaults. This involves analyzing historical loan data, including applicant demographics, credit history, and repayment patterns, to predict the likelihood of future loan repayments. Another example would be a marketing team predicting customer churn using machine learning models trained on past customer behavior.
Prescriptive Analytics Techniques
Prescriptive analytics goes beyond prediction by recommending actions to optimize outcomes. This often involves using optimization models, simulation, and decision support systems. For example, a logistics company could use prescriptive analytics to optimize its delivery routes, minimizing transportation costs and delivery times. This might involve using algorithms that consider factors such as traffic patterns, delivery deadlines, and vehicle capacity to determine the most efficient route for each delivery. Another application would be in finance, where portfolio optimization models help investors to maximize returns while minimizing risk.
Comparison of Business Analytics Techniques
The following table compares three key business analytics techniques: descriptive, predictive, and prescriptive analytics.
Technique Name | Description | Data Requirements | Typical Applications |
---|---|---|---|
Descriptive Analytics | Summarizes past data to understand what happened. | Historical data, often large datasets. | Sales reporting, web analytics, customer segmentation. |
Predictive Analytics | Forecasts future outcomes based on historical data and statistical models. | Historical data, potentially including external data sources. | Customer churn prediction, fraud detection, risk assessment. |
Prescriptive Analytics | Recommends actions to optimize outcomes based on predictions and optimization models. | Historical data, predictive models, and business rules. | Supply chain optimization, resource allocation, personalized recommendations. |
Examples of Business Analytics in Action: Which Of These Are Examples Of Business Analytics
Business analytics empowers organizations across diverse sectors to make data-driven decisions, improving efficiency and profitability. By leveraging descriptive, predictive, and prescriptive techniques, businesses gain valuable insights that inform strategic planning and operational improvements. The following examples illustrate the practical application of these analytical approaches.
Descriptive Business Analytics Examples
Descriptive analytics focuses on summarizing historical data to understand past performance. This understanding forms the foundation for more advanced analytical techniques. The following examples showcase the power of descriptive analytics in different industries.
- Retail: A major clothing retailer uses descriptive analytics to analyze sales data by product, region, and time period. This analysis reveals popular items, slow-moving inventory, and seasonal trends. This information informs inventory management, marketing campaigns, and pricing strategies, ultimately leading to increased sales and reduced waste. For instance, identifying a consistent underperformance of a particular clothing line in a specific geographic area allows for targeted marketing efforts or adjustments to the product line itself.
- Healthcare: Hospitals utilize descriptive analytics to track patient demographics, diagnoses, treatment outcomes, and length of stay. This data provides insights into hospital efficiency, identifies areas for improvement in patient care, and assists in resource allocation. For example, analyzing patient readmission rates helps pinpoint areas where post-discharge care could be improved, leading to better patient outcomes and reduced healthcare costs.
- Finance: Financial institutions use descriptive analytics to analyze customer transaction data, identifying spending patterns and potential fraud. This analysis helps in developing personalized financial products and services, improving risk management, and enhancing customer loyalty. For example, analyzing credit card transactions can identify unusual spending patterns that might indicate fraudulent activity, allowing for timely intervention and prevention of financial losses.
Predictive Business Analytics Examples
Predictive analytics uses historical data and statistical algorithms to forecast future outcomes. This allows businesses to anticipate trends and make proactive decisions. Two examples illustrate the value of predictive analytics in improving business decision-making.
- Marketing: An e-commerce company employs predictive analytics to predict customer churn. By analyzing customer behavior, purchase history, and engagement metrics, the company can identify customers at high risk of churning. This allows for targeted retention campaigns, such as personalized offers or discounts, to retain valuable customers and reduce customer churn rate. For example, a model might predict that customers who haven’t made a purchase in the last six months and have low website engagement are at high risk of churning.
- Supply Chain: A manufacturing company uses predictive analytics to forecast demand for its products. By analyzing historical sales data, seasonality, economic indicators, and other relevant factors, the company can accurately predict future demand. This enables optimized inventory management, reducing stockouts and minimizing excess inventory costs. For instance, the company might predict a surge in demand for a particular product during the holiday season, allowing them to increase production and secure sufficient raw materials in advance.
Prescriptive Business Analytics: Optimizing Supply Chain Resource Allocation
Prescriptive analytics goes beyond prediction by recommending optimal actions to achieve specific business goals. Consider a hypothetical supply chain scenario involving a food manufacturer with multiple distribution centers. By using prescriptive analytics, the company can optimize the allocation of its resources – specifically, its fleet of trucks – to minimize transportation costs while ensuring timely delivery to all its retail partners. The model would consider factors such as distance between distribution centers and retail locations, truck capacity, delivery deadlines, and real-time traffic conditions. The output would be a recommended delivery schedule that minimizes fuel costs, reduces delivery times, and improves overall supply chain efficiency. This optimized allocation reduces operational costs and improves customer satisfaction.
Business Analytics Tools and Technologies
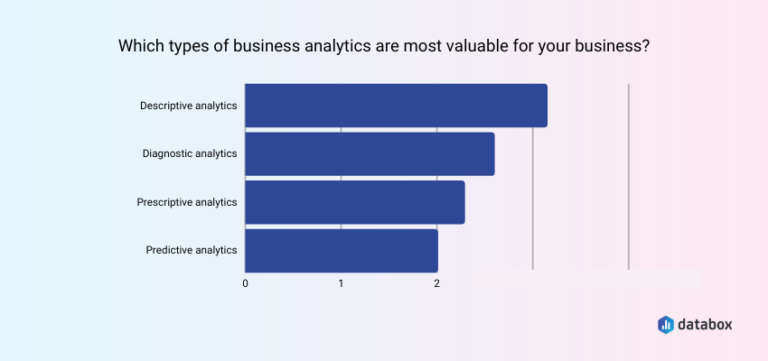
Effective business analytics relies heavily on the right tools and technologies to collect, process, analyze, and visualize data. The choice of tools often depends on the specific needs of the business, the size of the data sets, and the technical expertise available. A robust technology stack is crucial for extracting actionable insights and driving informed decision-making.
The selection of appropriate business analytics tools and technologies is a critical step in building a successful analytics program. Choosing the right tools depends on various factors including the size and type of data, the complexity of the analysis, the budget, and the technical expertise of the team. A well-integrated suite of tools can significantly improve efficiency and accuracy in deriving insights.
Commonly Used Business Analytics Software and Platforms
Many software platforms and tools facilitate various aspects of business analytics. These range from comprehensive suites offering a broad range of functionalities to specialized tools focused on specific tasks such as data visualization or predictive modeling. Selecting the right tools is crucial for efficient and effective analysis.
- Tableau: A leading data visualization and business intelligence platform known for its user-friendly interface and powerful analytical capabilities. It allows users to create interactive dashboards and reports to easily explore and share insights.
- Power BI: Microsoft’s business analytics service that provides interactive visualizations and business intelligence capabilities with an emphasis on integration with other Microsoft products. It allows users to connect to various data sources, create reports, and share dashboards.
- Qlik Sense: A data visualization and business intelligence platform known for its associative data exploration capabilities. It enables users to easily explore relationships between different data points and uncover hidden insights.
- R: A powerful open-source programming language and environment for statistical computing and graphics. It offers a vast library of packages for various statistical analyses, making it a popular choice for advanced analytics.
- Python: Another popular open-source programming language with extensive libraries (like Pandas, NumPy, and Scikit-learn) for data manipulation, analysis, and machine learning. It’s highly versatile and widely used in data science and business analytics.
- SAS: A comprehensive analytics suite offering a wide range of tools for data management, statistical analysis, and business intelligence. It’s often used in large enterprises for complex analytics projects.
- SPSS: A statistical software package commonly used for statistical analysis, including descriptive statistics, regression analysis, and hypothesis testing. It is known for its user-friendly interface and extensive statistical capabilities.
Data Visualization Tools: Tableau and Power BI
Data visualization is crucial for communicating complex data insights effectively. Two popular tools, Tableau and Power BI, offer distinct strengths in this area.
- Tableau: Tableau excels in creating visually appealing and interactive dashboards. Its drag-and-drop interface makes it relatively easy to build complex visualizations, even for users without extensive coding experience. Tableau’s strength lies in its ability to quickly transform raw data into compelling narratives, allowing for effective communication of key findings to both technical and non-technical audiences. For instance, a sales team might use Tableau to create a dashboard showing sales trends by region, product, and sales representative, allowing them to quickly identify areas for improvement.
- Power BI: Power BI integrates seamlessly with other Microsoft products, making it a convenient choice for organizations already using the Microsoft ecosystem. It offers robust data connectivity and allows for real-time data updates, providing dynamic and up-to-the-minute insights. A marketing team, for example, could use Power BI to track campaign performance in real-time, visualizing key metrics such as click-through rates, conversion rates, and cost per acquisition, enabling immediate adjustments to optimize campaigns.
Data Quality and its Impact on Business Analytics Results
The accuracy and reliability of business analytics results are directly dependent on the quality of the underlying data. Poor data quality can lead to flawed analyses, inaccurate predictions, and ultimately, poor decision-making. Data quality encompasses several aspects, including accuracy, completeness, consistency, timeliness, and validity.
Data cleansing and preprocessing are crucial steps to ensure data quality. This involves identifying and correcting errors, handling missing values, and transforming data into a consistent format suitable for analysis. For example, inconsistencies in data entry (e.g., using different formats for dates or addresses) can significantly impact the accuracy of analysis. Without proper data cleansing, these inconsistencies can lead to misleading results and inaccurate conclusions. Investing in robust data governance procedures and implementing data quality checks throughout the data lifecycle is essential for producing reliable and trustworthy business analytics results.
Ethical Considerations in Business Analytics
The increasing reliance on data and algorithms in business decision-making necessitates a thorough examination of the ethical implications. Business analytics, while offering powerful tools for optimization and growth, also presents potential risks if not approached responsibly. Understanding and mitigating these risks is crucial for maintaining trust, ensuring fairness, and upholding legal compliance.
Data privacy and algorithmic bias are among the most significant ethical challenges. The potential for misuse of sensitive information and the creation of discriminatory outcomes requires a proactive and ethical framework for the development and deployment of business analytics solutions. This section explores these challenges and proposes strategies for responsible data handling and algorithmic design.
Data Privacy and Security
Protecting user data is paramount in business analytics. The collection, storage, and use of personal information must adhere to strict privacy regulations, such as GDPR and CCPA. Data breaches can lead to significant financial losses, reputational damage, and legal repercussions. Robust security measures, including encryption, access controls, and regular security audits, are essential to safeguard sensitive data. Furthermore, organizations must be transparent with users about how their data is being collected, used, and protected, obtaining explicit consent whenever necessary. Implementing anonymization or pseudonymization techniques can help mitigate privacy risks while still allowing for valuable data analysis. For example, replacing personally identifiable information (PII) like names and addresses with unique identifiers prevents direct identification while preserving the integrity of the dataset for analytical purposes. Failure to adequately protect data can result in severe penalties and erosion of public trust.
Algorithmic Bias and Fairness
Algorithms used in business analytics are trained on data, and if that data reflects existing societal biases, the resulting algorithms can perpetuate and even amplify those biases. This can lead to unfair or discriminatory outcomes, such as biased loan applications, discriminatory hiring practices, or inaccurate risk assessments. For example, an algorithm trained on historical hiring data might inadvertently discriminate against certain demographic groups if past hiring practices were already biased. To mitigate algorithmic bias, organizations must carefully examine their data for biases, employ techniques to detect and mitigate bias in algorithms, and regularly audit the fairness of their analytical models. Transparency in algorithmic decision-making is also crucial, allowing for scrutiny and accountability. Employing diverse teams in the development and implementation of these systems can help identify and address potential biases before they become entrenched in the algorithms.
Responsible Use of Data and Algorithms in Decision-Making
The responsible use of data and algorithms in decision-making requires a multi-faceted approach. This includes establishing clear ethical guidelines, implementing robust data governance policies, and fostering a culture of ethical awareness within the organization. Decision-makers should understand the limitations of the data and algorithms and avoid over-reliance on automated systems. Human oversight and critical evaluation of analytical results are essential to ensure responsible decision-making. Furthermore, organizations should prioritize explainability and transparency in their analytical processes, enabling stakeholders to understand how decisions are made and to identify potential biases or errors. A well-defined ethical framework should guide the entire analytics lifecycle, from data collection to decision implementation, ensuring responsible and ethical use of data and algorithms throughout.
The Role of Business Analytics in Strategic Decision-Making
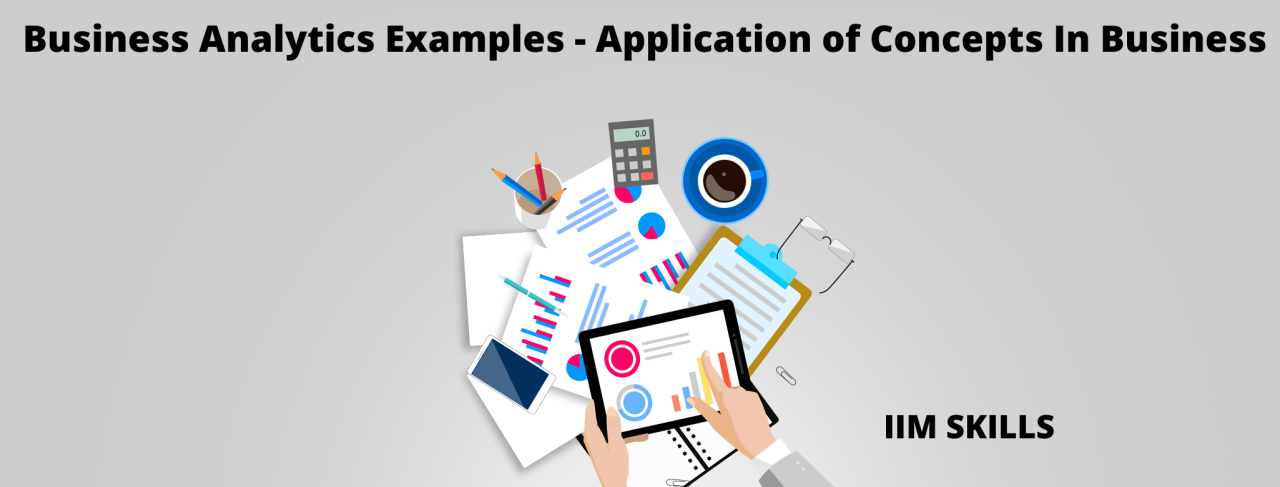
Business analytics plays a pivotal role in transforming raw data into actionable insights, empowering organizations to make informed strategic decisions and achieve sustainable competitive advantage. It moves beyond simple reporting, providing a predictive and prescriptive lens to navigate complex business landscapes and capitalize on emerging opportunities. This allows businesses to not only react to market changes but to proactively shape their future.
Business analytics supports strategic planning and execution by providing data-driven evidence to validate assumptions, refine strategies, and measure the effectiveness of implemented initiatives. Instead of relying on intuition or guesswork, organizations can leverage analytical models to forecast future trends, assess risks, and optimize resource allocation. This data-driven approach enhances the accuracy and precision of strategic planning, leading to better outcomes and reduced uncertainty.
Supporting Strategic Planning and Execution
Strategic planning relies heavily on accurate forecasting and risk assessment. Business analytics provides the tools to build robust predictive models using historical data, market trends, and economic indicators. For example, a retail company can utilize time-series analysis to forecast seasonal demand, enabling optimized inventory management and preventing stockouts or overstocking. Similarly, scenario planning, a technique facilitated by business analytics, allows organizations to explore the potential impact of various external factors (e.g., changes in regulations, competitor actions) on their business, enabling proactive mitigation strategies. Post-implementation, analytics helps track key performance indicators (KPIs) against established targets, allowing for mid-course corrections and continuous improvement.
Identifying New Market Opportunities
Business analytics enables the identification of untapped market segments and emerging trends through sophisticated data mining and market research techniques. By analyzing customer demographics, purchasing behavior, and online interactions, companies can identify unmet needs and develop targeted marketing campaigns. For instance, a telecommunications company might use customer segmentation analysis to identify a group of users with specific needs not currently being met by their existing product offerings. This insight can then be used to develop new products or services, capturing a previously untapped market share. Furthermore, sentiment analysis of social media data can reveal customer opinions and preferences, providing valuable feedback for product development and brand management.
Improving Operational Efficiency
Business analytics offers significant potential for enhancing operational efficiency across various departments. By identifying bottlenecks, inefficiencies, and areas for improvement, organizations can streamline processes and optimize resource utilization. For example, a manufacturing company can use process mining to analyze the flow of materials and identify areas where production time is unnecessarily extended. This allows for targeted interventions, such as process re-engineering or automation, leading to reduced production costs and increased output. Similarly, predictive maintenance, enabled by analytics, allows companies to anticipate equipment failures and schedule maintenance proactively, minimizing downtime and reducing maintenance costs. Analyzing logistics data can optimize delivery routes, reducing transportation expenses and improving delivery times.
Illustrative Examples

Visual representations are crucial for understanding complex business analytics concepts. They transform abstract ideas into easily digestible formats, fostering better comprehension and communication among stakeholders. This section provides detailed descriptions of visual representations illustrating key aspects of business analytics workflows, data relationships, and the integration of various analytical techniques.
Business Analytics Project Workflow, Which of these are examples of business analytics
This visual would be a flowchart, depicting a linear progression through the stages of a typical business analytics project. The first stage, “Problem Definition,” would be a box at the top, branching down to “Data Collection,” which could then split into two sub-processes: “Internal Data Acquisition” and “External Data Acquisition.” These would merge into “Data Cleaning and Preparation,” followed by “Exploratory Data Analysis (EDA),” which would lead to “Model Selection and Development.” The next step, “Model Validation and Testing,” would feed into “Deployment and Monitoring,” the final stage at the bottom of the flowchart. Feedback loops would be shown from “Monitoring” back to “Model Selection and Development” and “Data Collection,” highlighting the iterative nature of the process. Each box would contain a brief description of the activities involved in that stage.
Relationship Between Data, Information, and Insights
This visual would be a pyramid. At the base, the widest part, would be “Data,” representing the raw, unorganized facts and figures. The next level, narrower than the base, would be “Information,” depicting data that has been organized, structured, and processed to provide context. Finally, at the apex of the pyramid, would be “Insights,” representing the actionable knowledge and understanding derived from analyzing the information. Arrows would connect each level, illustrating the transformation of data into information and ultimately into valuable insights. Examples of each level could be included: “Data” could be sales figures, “Information” could be average monthly sales, and “Insights” could be a prediction of future sales based on identified trends.
Contribution of Different Business Analytics Techniques
This visual would be a Venn diagram showing the overlap and interplay of different business analytics techniques. Three overlapping circles could represent “Descriptive Analytics,” “Predictive Analytics,” and “Prescriptive Analytics.” Within each circle, specific techniques would be listed: “Descriptive Analytics” could include data aggregation, data mining, and reporting; “Predictive Analytics” could include regression analysis, machine learning, and forecasting; and “Prescriptive Analytics” could include optimization, simulation, and decision support systems. The overlapping areas would highlight how these techniques work together. For example, the overlap between “Descriptive” and “Predictive” could illustrate how descriptive analytics provides the foundation for predictive models. The area where all three circles intersect would represent the holistic understanding achieved by combining all techniques to solve a business problem. A central area could illustrate the overall business problem being addressed. For example, understanding customer churn could be the central problem, with each technique contributing different perspectives and solutions.