Why resolution is important in business? The answer lies in its transformative power across every facet of an organization. From sharper decision-making fueled by granular data insights to profoundly enhanced customer understanding and proactive risk management, high-resolution data empowers businesses to thrive in today’s competitive landscape. This detailed exploration reveals how superior data resolution translates into tangible advantages, impacting everything from operational efficiency to innovation and market leadership.
Imagine the difference between a blurry photograph and a crystal-clear image. That’s the essence of data resolution in business. Low-resolution data, like the blurry photo, provides a vague, incomplete picture, leading to flawed decisions and missed opportunities. High-resolution data, on the other hand, offers a detailed, precise understanding, enabling informed choices and strategic advantages. This article delves into the multifaceted impact of high-resolution data, demonstrating its crucial role in achieving business success.
Improved Decision-Making
High-resolution data is the cornerstone of effective business decision-making. The ability to analyze detailed, granular information allows businesses to identify trends, patterns, and anomalies that would be invisible with less precise data. This leads to more accurate predictions, better resource allocation, and ultimately, improved business outcomes. Conversely, relying on low-resolution data can lead to costly mistakes and missed opportunities.
High-resolution data facilitates informed business decisions by providing a comprehensive and nuanced understanding of various aspects of the business. This allows for a more accurate assessment of risks and opportunities, leading to strategic choices that are better aligned with the company’s goals. For example, detailed customer segmentation based on purchasing behavior, demographics, and preferences enables targeted marketing campaigns that yield higher conversion rates than generic approaches. Similarly, analyzing granular sales data can reveal regional variations in demand, allowing for optimized inventory management and supply chain strategies.
Examples of Decision-Making with Low vs. High-Resolution Data
Consider a hypothetical scenario where a clothing retailer is planning its next season’s collection. Using low-resolution data, such as overall sales figures for the past year, the retailer might conclude that a particular style of dress is underperforming and decide to discontinue it. However, high-resolution data, including sales figures broken down by region, age group, and specific dress color, might reveal that the dress is highly popular among a specific demographic in a certain region. This nuanced insight would lead the retailer to maintain the dress, perhaps adjusting its marketing strategy to target the identified segment more effectively, instead of discontinuing a potentially profitable product line.
Another example involves a food manufacturer using low-resolution data for market research. They might conduct a broad survey asking about overall satisfaction with their product, resulting in generally positive but unspecific feedback. With high-resolution data, they could analyze detailed consumer reviews, social media mentions, and purchasing patterns to identify specific aspects of the product that need improvement. This detailed analysis might reveal that, while the product is generally well-liked, a particular flavor variant is unpopular or that packaging design is a point of dissatisfaction, allowing for targeted improvements rather than broad, potentially ineffective changes.
Market Research and Strategic Alteration
A company specializing in online education initially targeted its courses broadly to a wide demographic. Their initial market research, using low-resolution data like overall website traffic and course enrollment numbers, suggested a need for broader marketing efforts. However, after investing in high-resolution data analytics, including detailed student profiles, learning patterns, and feedback, they discovered that a specific niche within the broader demographic—working professionals seeking career advancement—showed significantly higher engagement and completion rates. This discovery led to a strategic shift, focusing marketing efforts and course development on this niche market. This resulted in a dramatic increase in student retention and overall revenue.
Impact of Data Resolution on Key Business Metrics
Metric | Low-Resolution Data Impact | High-Resolution Data Impact | Example |
---|---|---|---|
Sales | Inaccurate sales forecasting, leading to stockouts or overstocking. | Precise sales predictions, optimized inventory management, increased revenue. | A retailer misjudges demand and runs out of a popular item (low resolution) vs. accurately predicting demand and maximizing sales (high resolution). |
Customer Retention | High churn rate due to inability to identify customer needs and preferences. | Targeted customer engagement, personalized offers, improved loyalty programs. | A company loses customers due to generic marketing (low resolution) vs. retaining customers through personalized experiences (high resolution). |
Market Share | Missed opportunities to capture market segments, slower growth. | Identification of underserved markets, strategic targeting, increased market penetration. | A company fails to identify a growing niche market (low resolution) vs. successfully capturing market share in a specific segment (high resolution). |
Marketing ROI | Low return on marketing investment due to ineffective targeting. | High return on investment through precise targeting and optimized campaigns. | A company spends heavily on generic ads with poor results (low resolution) vs. achieving high ROI through targeted advertising (high resolution). |
Enhanced Customer Understanding
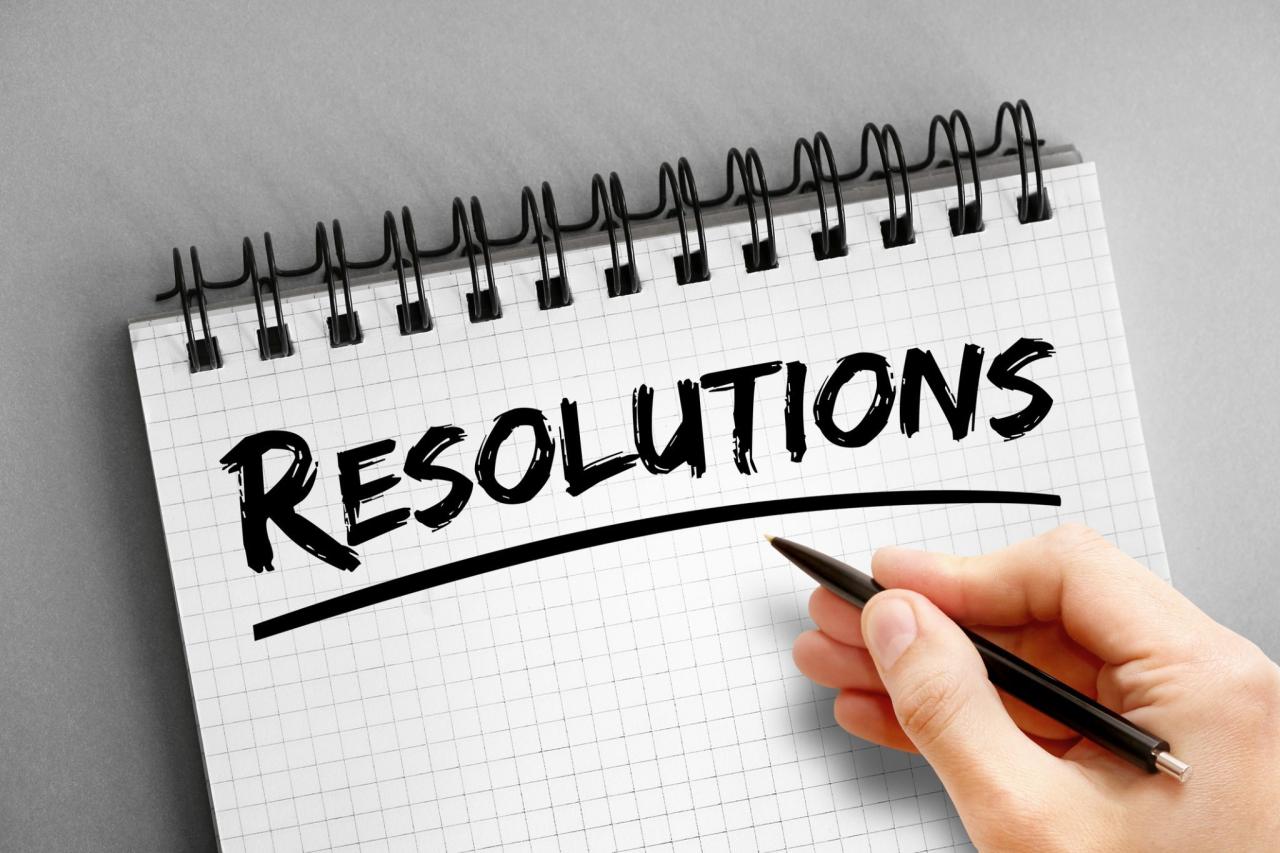
High-resolution customer data is the cornerstone of modern, effective marketing. It allows businesses to move beyond broad generalizations and engage with individual customers on a much more personal and relevant level. This granular understanding fuels more effective targeting, personalization, and ultimately, increased customer loyalty and revenue. The ability to see the complete picture of a customer, rather than fragmented pieces of information, is what truly transforms the customer experience.
Detailed customer data significantly improves personalization and targeting efforts by enabling businesses to tailor their messaging and offers to resonate specifically with individual customer needs and preferences. Instead of sending generic emails to a massive list, businesses can segment their audience and deliver highly targeted content. This targeted approach leads to higher engagement rates, improved conversion rates, and ultimately, a better return on investment (ROI) for marketing campaigns.
Leveraging High-Resolution Customer Data for Effective Marketing Campaigns, Why resolution is important in business
Netflix’s recommendation engine is a prime example of high-resolution data in action. By meticulously tracking viewing history, ratings, and even viewing time, Netflix creates incredibly accurate personalized recommendations. This granular level of data allows them to suggest shows and movies that are highly likely to appeal to each individual user, leading to increased viewing time and subscriber retention. Similarly, Amazon uses detailed purchase history, browsing behavior, and product reviews to personalize product recommendations and targeted advertising, resulting in increased sales and customer engagement. These companies demonstrate the power of utilizing high-resolution data to create highly effective marketing campaigns that resonate deeply with individual customers.
Benefits of High-Resolution Data for Customer Segmentation and Personalized Recommendations
High-resolution customer data enables sophisticated customer segmentation, allowing businesses to divide their audience into smaller, more homogenous groups based on shared characteristics, behaviors, and preferences. This allows for highly targeted messaging and offers, maximizing impact and minimizing wasted resources. For example, a clothing retailer might segment customers based on age, style preferences, purchase history, and browsing behavior to create targeted email campaigns promoting relevant products. Furthermore, this detailed understanding facilitates the creation of personalized recommendations, mirroring the success of Netflix and Amazon. By understanding individual customer preferences, businesses can offer products and services that directly address their needs, increasing the likelihood of purchase and fostering customer loyalty.
Types of High-Resolution Customer Data and Their Applications
Understanding the various types of high-resolution customer data and their potential applications is crucial for maximizing its benefits. The more comprehensive the data, the more refined the customer understanding and the more effective the personalization efforts.
- Demographic Data: Age, gender, location, income, education level. Used for targeted advertising and product development.
- Behavioral Data: Website activity, purchase history, app usage, social media engagement. Used for personalized recommendations and retargeting campaigns.
- Psychographic Data: Lifestyle, interests, values, attitudes. Used for creating highly targeted and resonant marketing messages.
- Transactional Data: Purchase history, payment methods, order frequency. Used for predicting future purchases, identifying high-value customers, and personalizing offers.
- Geolocation Data: Location data from mobile devices or IP addresses. Used for location-based marketing and personalized offers.
Effective Risk Management
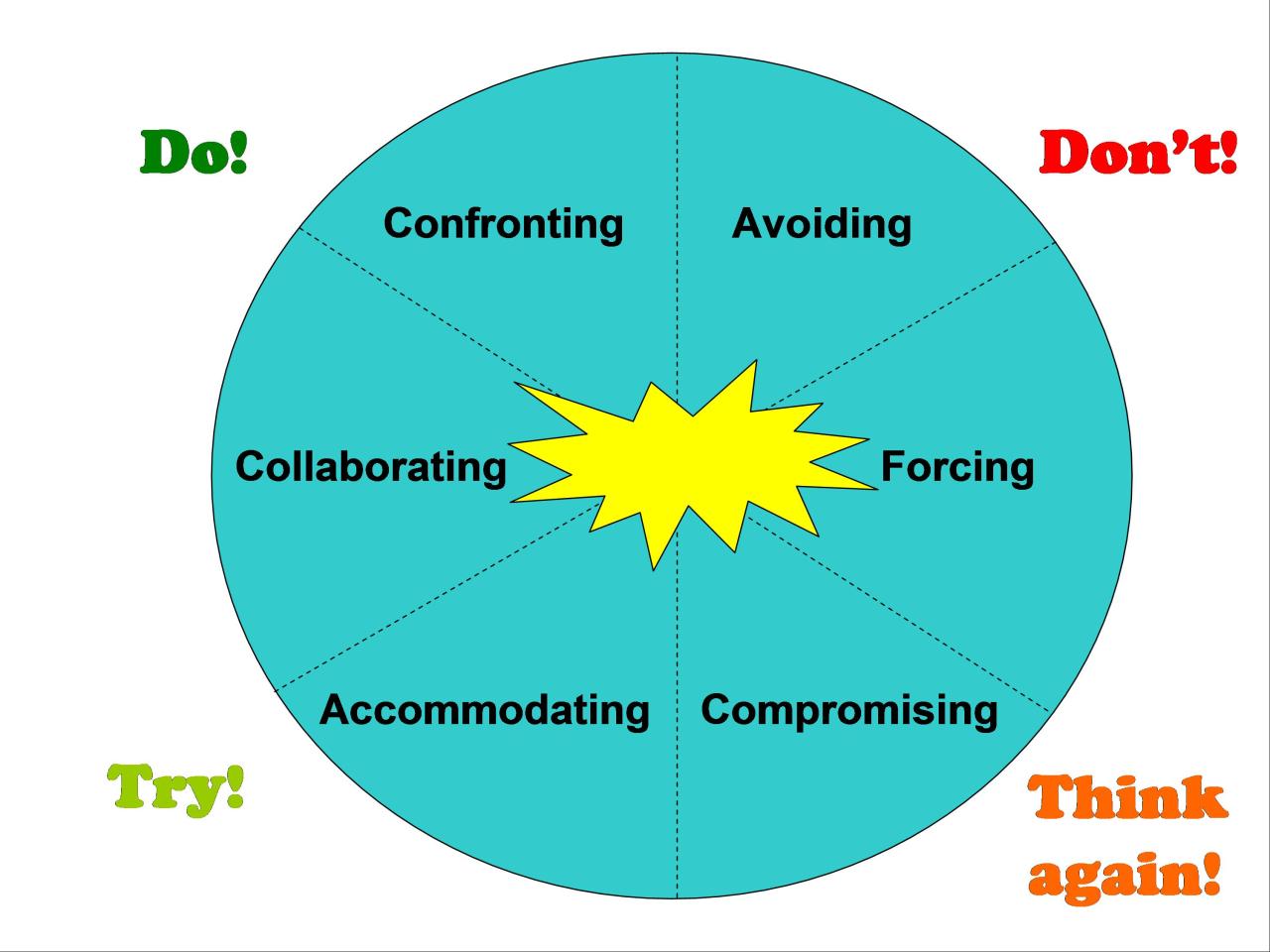
High-resolution data significantly enhances a business’s ability to proactively manage and mitigate risks. By providing a granular view of operations and market dynamics, it allows for a more precise assessment of potential threats and the development of targeted mitigation strategies. This contrasts sharply with low-resolution data, which often provides an incomplete and potentially misleading picture, hindering effective risk management.
High-Resolution Data in Proactive Risk Identification and Mitigation
High-resolution data, encompassing detailed and real-time information across various business functions, allows for the identification of subtle patterns and anomalies that might otherwise go unnoticed. This proactive approach allows businesses to address potential problems before they escalate into major crises. For instance, analyzing high-resolution sales data might reveal a sudden dip in a specific product line in a particular region, indicating a potential problem with distribution, marketing, or product quality. This early warning allows for immediate investigation and corrective action, preventing further losses.
Comparison of Risk Assessment Strategies
Employing low-resolution data for risk assessment often leads to generalized and potentially inaccurate conclusions. For example, relying solely on aggregate sales figures might mask regional variations or specific customer segments experiencing difficulties. In contrast, high-resolution data enables a more nuanced understanding of risk, allowing for the identification of specific vulnerabilities and the tailoring of mitigation strategies to address them effectively. A high-resolution approach might involve analyzing individual customer transactions, social media sentiment, and supply chain data to pinpoint the exact source of a problem.
Real-Time Data and Operational Efficiency
Real-time, high-resolution data streams significantly improve operational efficiency and minimize downtime. Imagine a manufacturing facility utilizing sensors to monitor equipment performance with high-resolution data. An anomaly in a machine’s vibration pattern, imperceptible with less granular data, can be detected instantly, triggering preventative maintenance before a complete failure occurs. This prevents costly production stoppages and ensures consistent output. Similarly, real-time tracking of inventory levels prevents stockouts and ensures timely replenishment.
Examples of Risk Mitigation with High-Resolution Data
Risk | Low-Resolution Data Approach | High-Resolution Data Approach | Mitigation Improvement |
---|---|---|---|
Supply Chain Disruption | Aggregate inventory data showing overall low stock levels. | Real-time tracking of individual components and their location, identifying specific bottlenecks. | Faster identification of disrupted supply points, allowing for quicker alternative sourcing and minimized production delays. |
Customer Churn | Overall customer satisfaction scores showing a slight decline. | Analysis of individual customer interactions, identifying specific pain points and dissatisfaction triggers. | Targeted interventions to address specific customer concerns, improving retention rates. |
Fraudulent Activity | Aggregate financial reports showing unusual spending patterns. | Real-time monitoring of individual transactions, flagging suspicious activities based on predefined rules and machine learning algorithms. | Immediate detection and prevention of fraudulent transactions, minimizing financial losses. |
Equipment Malfunction | Periodic equipment inspections revealing general wear and tear. | Continuous monitoring of equipment performance through high-resolution sensor data, detecting anomalies before failure. | Preventative maintenance scheduling, minimizing downtime and extending equipment lifespan. |
Streamlined Operations
High-resolution data empowers businesses to optimize their operational processes and significantly boost productivity. By providing a granular view of various aspects of the business, it enables more accurate forecasting, efficient resource allocation, and proactive identification of bottlenecks. This ultimately translates into cost savings, improved efficiency, and a stronger competitive edge.
High-resolution data facilitates the identification of previously unseen patterns and anomalies within operational workflows. This granular level of detail allows for the pinpoint detection of inefficiencies, enabling targeted interventions to streamline processes and eliminate waste. For example, analyzing high-resolution sensor data from manufacturing equipment can reveal subtle variations in performance that might otherwise go unnoticed, leading to preventative maintenance and avoiding costly downtime.
High-Resolution Data in Operational Efficiency Across Industries
The benefits of high-resolution data extend across diverse sectors. In logistics, real-time tracking data provides unparalleled visibility into the movement of goods, allowing for optimized routing and faster delivery times. High-resolution satellite imagery aids precision agriculture by providing detailed insights into crop health, enabling farmers to tailor irrigation and fertilization strategies for maximum yield. In the healthcare industry, high-resolution medical imaging allows for more accurate diagnoses and personalized treatment plans, improving patient outcomes and reducing healthcare costs.
Operational Inefficiencies Due to Lack of High-Resolution Data
Consider a large retail chain lacking a robust inventory management system with high-resolution data. Without accurate, real-time data on stock levels, the company might experience stockouts of popular items, leading to lost sales and dissatisfied customers. Conversely, overstocking of slow-moving items ties up capital and increases storage costs. The lack of granular data on customer preferences and purchasing patterns further exacerbates the problem, leading to inefficient inventory allocation and ultimately impacting profitability. The absence of high-resolution data in this scenario resulted in significant operational inefficiencies and increased costs associated with lost sales, storage, and potentially even waste disposal of outdated inventory.
Advantages of High-Resolution Data in Supply Chain Management and Inventory Control
High-resolution data offers several advantages for effective supply chain management and inventory control. Understanding the importance of this data is crucial for achieving operational excellence.
- Improved Forecasting Accuracy: High-resolution sales data allows for more accurate demand forecasting, minimizing stockouts and reducing waste from overstocking.
- Optimized Inventory Levels: Real-time visibility into inventory levels enables just-in-time inventory management, reducing storage costs and minimizing the risk of obsolescence.
- Enhanced Supply Chain Visibility: Tracking goods throughout the supply chain provides real-time insights into potential disruptions, allowing for proactive mitigation strategies.
- Reduced Lead Times: Optimized processes and accurate forecasting contribute to shorter lead times, improving customer satisfaction and reducing operational costs.
- Better Resource Allocation: Data-driven insights enable efficient allocation of resources, including transportation, warehousing, and personnel, optimizing overall efficiency.
Competitive Advantage
High-resolution data offers a significant competitive advantage in today’s data-driven business environment. The ability to collect, analyze, and act upon granular, precise information allows businesses to make more informed decisions, optimize operations, and ultimately, outperform their rivals. This advantage stems from the richer insights gleaned from high-resolution data, enabling a more nuanced understanding of customer behavior, market trends, and operational efficiencies.
Access to and effective use of high-resolution data provides a powerful competitive edge by enabling businesses to develop more targeted strategies, personalize customer experiences, and optimize resource allocation. This translates to increased efficiency, reduced costs, and ultimately, higher profitability. Conversely, companies lacking access to or the expertise to utilize high-resolution data may find themselves at a significant disadvantage, struggling to keep pace with more agile and data-savvy competitors.
High-Resolution Data in Action
Several companies have successfully leveraged high-resolution data to gain a significant competitive edge. For example, Netflix uses high-resolution data on user viewing habits to personalize recommendations, improving customer satisfaction and reducing churn. This granular data allows them to predict viewing trends, inform content creation decisions, and optimize their content delivery infrastructure. Similarly, Amazon uses high-resolution data on customer purchases and browsing behavior to personalize product recommendations, optimize inventory management, and target advertising campaigns with exceptional precision. These examples demonstrate how high-resolution data, when effectively utilized, can lead to a substantial increase in revenue and market share.
Data Security and Privacy Considerations
The use of high-resolution data necessitates robust data security and privacy measures. Given the sensitive nature of this information, companies must implement stringent protocols to protect data from unauthorized access, breaches, and misuse. This includes adhering to relevant data privacy regulations, such as GDPR and CCPA, and investing in advanced security technologies like encryption and access control systems. Failing to prioritize data security can lead to significant financial losses, reputational damage, and legal repercussions. A strong commitment to data security and privacy is therefore crucial for responsible and sustainable use of high-resolution data.
Hypothetical Scenario: Market Share Dominance Through Data Resolution
Imagine a hypothetical scenario involving two competing grocery chains: “Grocer A” and “Grocer B.” Grocer A invests heavily in high-resolution data collection, utilizing loyalty programs and advanced analytics to understand individual customer preferences at a granular level – down to specific product brands, purchase frequencies, and even preferred shopping times. Grocer B relies on aggregated sales data and broader market trends. Grocer A uses its high-resolution data to personalize promotions, optimize product placement, and even tailor store layouts to individual customer preferences. This results in increased customer loyalty, higher sales conversions, and ultimately, a significant increase in market share, outperforming Grocer B which lacks the same level of data granularity and insights. This scenario highlights the transformative potential of high-resolution data in achieving a commanding market position.
Innovation and New Product Development: Why Resolution Is Important In Business

High-resolution data plays a pivotal role in driving innovation and new product development. By providing a granular understanding of customer behavior, market trends, and product performance, high-resolution data empowers businesses to make informed decisions, reducing risk and increasing the likelihood of success. This detailed information allows for the creation of products and services that precisely meet customer needs and preferences, ultimately leading to a stronger competitive advantage.
High-resolution data informs the development of new products and services in several key ways. Firstly, it allows for the identification of previously unseen patterns and trends in customer behavior. This detailed understanding can reveal unmet needs and opportunities for innovation that might be missed with less granular data. Secondly, it enables businesses to precisely target specific customer segments with tailored products and services, maximizing impact and return on investment. Finally, the ability to track product performance in real-time allows for rapid iteration and improvement, ensuring the product remains relevant and competitive.
High-Resolution Data in New Product Development
Analyzing high-resolution data, such as detailed customer purchase history, website browsing behavior, and social media interactions, enables businesses to identify unmet needs and emerging trends. For instance, a company analyzing customer purchase data might discover a high demand for a specific product feature that wasn’t previously offered. This insight can then be used to develop a new product or improve an existing one to meet this previously unrecognized need. Similarly, analyzing website analytics with high resolution can reveal which features customers are using most frequently and which are being neglected, providing valuable insights for product refinement.
Examples of Successful Product Innovations Driven by High-Resolution Data
Netflix’s recommendation engine is a prime example of how high-resolution data analysis fuels innovation. By meticulously tracking user viewing habits, including the time spent watching specific shows and genres, Netflix can personalize recommendations with remarkable accuracy. This personalized experience has been instrumental in their success and has significantly improved user engagement and retention. Similarly, Amazon leverages vast amounts of high-resolution data on customer purchases, browsing history, and reviews to inform product development and personalize the customer shopping experience. Their recommendation system and targeted advertising campaigns are direct results of this data-driven approach.
High-Resolution Customer Feedback in Product Design Refinement
High-resolution customer feedback, obtained through surveys, focus groups, and online reviews, is crucial for refining product design and features. Instead of relying on broad, generalized feedback, high-resolution data allows businesses to understand the nuances of customer opinions. For example, analyzing detailed customer reviews of a particular product can pinpoint specific areas for improvement, such as usability issues or design flaws. This detailed feedback allows for targeted improvements, leading to a more refined and user-friendly product. By understanding the specific pain points and preferences of their customers, companies can create products that resonate deeply with their target market.
“Data is the new oil, but it’s useless unless you refine it.” – Clive Humby, data scientist and pioneer of customer relationship management (CRM)